
Frontier technologies are making algorithmic trading accessible to small traders: Nitesh Khandelwal, QuantInsti
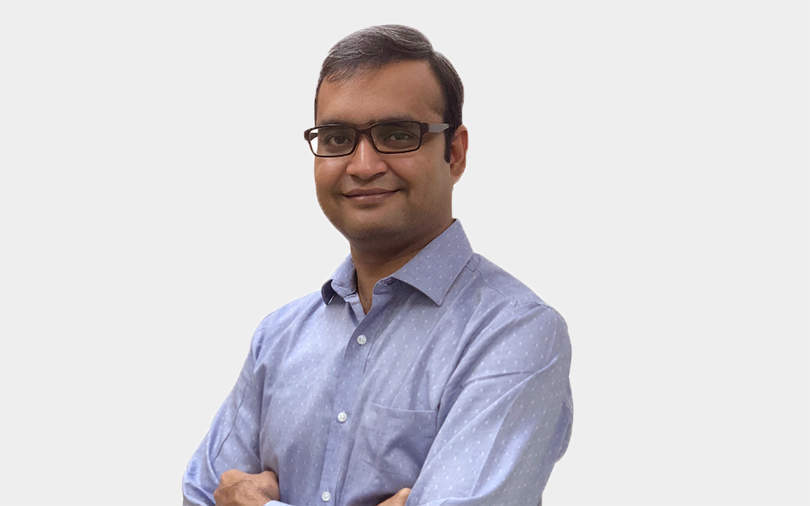

Back in the seventies, stock market traders realized they could create complex mathematical equations, input financial values and gain quicker insights to get an edge over fellow traders. This came to be known as algorithmic trading. It has become a tool of choice today for most big stock market brokerages that process high volumes.
What’s interesting is that fundamentals of algorithmic trading are similar to machine learning (ML) and artificial intelligence (AI). Both technologies build on algorithms, feed them data and make them better over time. No surprise then that algorithmic trading has found a long-term ally in data sciences, AI and ML, with the technologies not only helping to make trading simpler and easier, but also making it more accessible to small traders.
Nitesh Khandelwal, co-founder of QuantInsti, an algorithmic trading training institute that offers an executive programme in algorithmic trading (EPAT), has been closely following the growth of the trend and has been building algorithmic technology trading platforms since 2007, through ICICI Bank and iRage, one of the pioneers in the field of High Frequency Trading in India.

In an interview with TechCircle, Khandelwal spoke how the advent of computational power and AI/ML has led to an increase in the efficiency and accuracy in algorithmic trading and the role that SEBIs adoption of technologies has played in making all types of trading more efficient. He also sheds light on how technology has led to the democratization of algorithmic trading.
Edited excerpts:
Could you explain how algorithmic trading works?

Algorithmic trading is the use of algorithms to create, model, research and execute trading strategies. In most of the cases, these types of trading strategies include fully automated execution. Additionally, algorithms can also be used for managing portfolios, risk management, etc. Many people assume that algorithmic trading is the same as High Frequency Trading (HFT), which is not correct. The HFT strategies generally constitute a very high number of orders, high volume and low holding periods. It is true that all HFT strategies use algorithms, but not all algorithmic trading strategies are HFT.
How has it become faster and more efficient over the years?
The electronic trading era began in the 1970s. NYSE was the first exchange to allow heavy use of computers for trading in the late 1970s. This enabled traders to manually trade using computers from anywhere instead of physically going to the trading pit in the exchange. With the advent of computers and their applications, traders started to use algorithms that could place orders in an automated fashion. This was the start of algorithmic trading and it resulted in a drastic reduction in execution times.

Today, a HFT firm is able to send thousands of orders every second. The new-age systems are able to analyze the data, process and send out the orders within a few hundred nanoseconds. This means they are able to respond, or rather profit from any inefficiency in the market much faster, making the markets more efficient.
Not just that, technology has enabled the stock market exchanges to process and match orders much faster. Faster communication between the exchange and traders’ systems means lower uncertainty for the traders about the state of their orders. This assists the trading firms, especially the market-making firms, to quote much tighter bid-ask spreads. This has also helped the retail investors and traders by reducing the cost of transactions.
What are the technological pillars crucial to algorithmic trading?

Algorithmic trading is dependent on many technologies. A couple among those are FPGA (field programmable gate array) and microwave -- a communications system that uses a beam of radio waves in the microwave frequency range to transmit video, audio, or data between two locations.
FPGA, the speed game to reach the exchange fastest, moved from the software domain to hardware. The HFT firms that are focused on arbitrage and market making strategies are now exploring or already using Field Programmable Gate Array (FPGA), both as a means to connect to the exchange (adapters) as well as for coding the strategies right in the hardware itself.
Firms involved in classic inter-exchange arbitrage strategies used to connect via optic fibre cables. But in most of the developed markets, microwave is replacing that as it gives a faster transmission speed compared to fibre. In some of the markets, LASER, which facilitates transmission at nearly the speed of light, is also being explored.

Both the above technologies are heating up the race to zero latency. But these technological changes are largely impacting only the ultra High Frequency Trading (HFT) firms who are primarily focused on risk-free arbitrage strategies, where speed is one of the few advantages that one can have.
How are emerging technologies making algorithmic trading accessible to small traders?
Some of the new technologies that are enabling algorithmic trading even for retail, individual traders, and investors include data sciences, the programming language Python, cloud computing and API trading.

With respect to data sciences, ML and AI are now becoming mainstream. Most of the industries, from automobile to ecommerce are actively using ML and AI applications. The financial markets are not far behind. Traders and investors are actively using ML techniques to come up with new sources of alpha from trading strategies, building and managing portfolios, enhancing risk management and for a host of other use cases.
While Python has been around since the early nineties, it has seen another level of penetration with the advent of data science. It is easier to learn, it’s free and open source and has lots of readymade packages or libraries that are very useful for data analysis. It is getting very popular in the financial markets as well.
Cloud infrastructure offered by the likes of Amazon AWS, Microsoft Azure and others are providing easy access to computing power and robust infrastructure on the go in a very affordable manner. What it means is that an individual trader does not have to let go of complex trading ideas for the lack of computational power, which was the case earlier.
Finally, the rising base of traders and investors who are skilled in analytics and programming has compelled most big brokerage firms to open up their trading systems for their users who can connect through various APIs including Python, REST and many others.
How has SEBI’s (Securities and Exchange Board of India) adoption of technology impacted algorithmic trading?
SEBI has been very proactive in the adoption of new technology in the financial markets and has facilitated a very smooth evolution of new paradigms in the Indian markets, including algorithmic trading.
SEBI came in with broad guidelines on algorithmic trading soon after it allowed the same. These guidelines were reasonably exhaustive and ensured the constructive growth of the industry. In fact, it is to the credit of SEBI and the Indian stock exchanges that flash crash occurrences have been contained much better than most of the other major markets. (The term flash crash comes from a trillion-dollar stock market crash in the United States, which started at 2:32 p.m. EDT on May 6th, 2010 and lasted for approximately 36 minutes).
SEBI has also been very proactive in addressing any threats or concerns that can come up due to any new technology. A few years back, it also came up with a white paper to look at the measures for strengthening the algorithmic trading framework, where consultations were taken from various market participants.
All these measures are definitely helping a healthy growth of algorithmic trading in the financial markets, which shall certainly help more people to go algo!