
How CRISIL is going cloud native, leveraging NLP in the next digitization phase
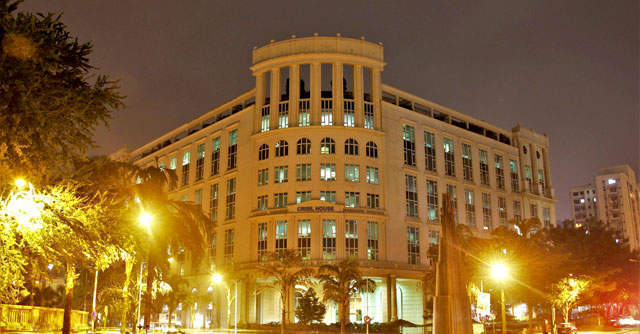

For S&P Global owned ratings agency CRISIL, the post Covid-19 operating strategy is going to be all about user side digitization, powered by microservices, natural language processing (NLP) tools and cloud-based data hosting models.
A prominent part of this strategy is a new portal that the Mumbai headquartered company plans to roll out to enable its clients -- micro, small and medium enterprises, large corporates and a host of financial institutions -- to track different parameters of functioning for both internal and external stakeholders.
In February this year, CRISIL completed the acquisition of US-based Greenwich Associates, a benchmark analytics firm that derives insights by talking externally to clients of customers. The acquisition helped CRISIL close the loop on capabilities from another prior acquisition, UK based Coalition, which gave the ratings agency to conduct benchmark analytics by talking to internal stakeholders such as CXOs.

“We thought why can’t we combine both these products and accelerate its development? Let's create a digital portal at the center where clients can look at both sides of the product,” CRISIL CIO Ramesh Lakshminarayanan told TechCircle.
The product, which is currently in second-level testing, is expected to be released this month as a web portal and a mobile application and will provide the firm’s clients with insights on how their organisations function internally and externally.
While CRISIL is primarily regarded as a ratings agency in India, what’s relatively less known about the company is that over the last few years it has diversified significantly into risk based advisory, risk based modelling and provides fundamental research for global clients.

The company is 90% data driven and began its digitization drive in 2018, which has continued with some key deployments in 2020, including the upcoming portal, and an accelerated migration to the hybrid cloud.
In the first phase of its digitization, the company consolidated all its data lying at different locations. The second step was to analyse and build technology applications to make that data easily consumable.
“Can we have mobile interfaces? Build digital portals? Can we provide API based feeds for data? Because these are fundamental to the digitization revolution,” said Lakshminarayanan.

The third phase, currently underway, is to propel the data into an application, starting out with basic business intelligence tools and moving on to deep research and analysis through machine learning and artificial intelligence solutions.
“We then look to input the research data into the ratings data, create models out of it and push the analysis back into the consumable front end application… That is how our digital strategy is slowly taking shape currently,” added Lakshminarayanan.
Transition from legacy applications to microservices and the cloud
Crisil had done a fair bit of work in building the data layer and re-architecting legacy applications that were built on .net and Java, which were moved to a microservices based Java framework called Java Spring Boot, as well as Google’s modern open source developer platform Angular.

“Over the last 14-15 months we have built around 8-10 microservices components such as workflow, rule engine, data mapping as a service, visualization tool kit, screen builder, python executor,” said Lakshminarayanan. This move ensures that the company does not have to build new applications from scratch.
Another big step was to move the data and analysis onto cloud native platforms. “We've done a fair bit of work in building these microservices led application platforms over the last one and a half years. In parallel, we also created a series of data science capabilities,” he added.
Watch: CRISIL CIO on the credit ratings agency’s pivot to microservices

CRISIL also uses machine learning to extract data from PDF and financial statements and interpret in a language that is easily consumable.
In the next step to map the data extracted, natural language processing methods were used. CRISIL is using machine learning on the data to create models. An example is the financial sensitivity model, which can proactively recognize (and decipher reasons for change in) companies with deteriorating financial health, help users take pre-emotive steps for optimal risk based pricing of debt by identifying potential stress points, as well as leverage CRISIL’s proprietary framework based on qualitative and quantitative parameters.
Read: Avoid these five anti-patterns when going platform-first

Moving forward, it aims to automate the writing of the analysis of the reports.
Covid-19 and business continuity
In the post Covid-19 world, Lakshminarayanan aims to bring collaborative tools such as Zoom, Cisco WebEx, email, chat engines from segregated entities onto a single, solid monolithic platform.
“...the whole idea of the business continuity planning(BCP) and disaster recovery(DR) running out of the cloud architecture, is one thing that Covid-19 has shown us,” he said.
He also plans to move more applications into cloud native environments. “The cloud move will easily take care of our DR, BCPS, remote management and other areas,” he said.
Another area CRISIL is working is the conversion of large sets of information, currently in the form of video recordings, market commentaries and analyst recordings, into data on top of which analytics can be run to derive insights. Additionally, there is a focus on natural language generation through which the company aims to extract data from interpreting financial statements and obtain analysis.
As part of its security strategy, CRISIL had teamed up with a deception technology startup that mimics physical networks to trap and block potential cybersecurity threats. The solution works by creating a mirage of the entire network, including exact folder topologies and naming conventions. The solution ensures that the real network is harder to recognise and can also alert the original servers on any incoming threat vectors.