
Fairness and bias in AI depend on how data is prepared: Sameep Mehta, IBM
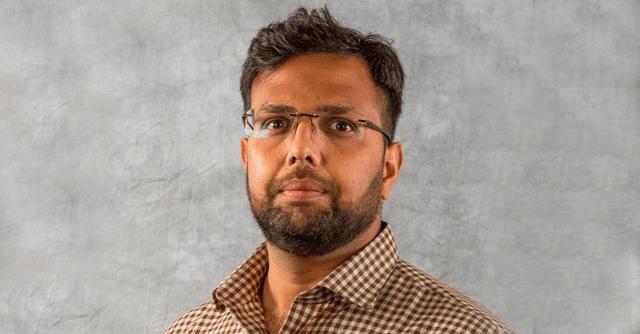

Most of the talent currently is focused on creating algorithms for AI and building software for its deployment, but a critically overlooked factor to the AI puzzle is how to ensure that the right data is fed into these systems. An IDC report in March stated that 60% of enterprise decisions will be taken based on AI inputs. In such a scenario, according to IBM Research India’s Data and AI platforms lead, Sameep Mehta, the most critical components are ensuring that AI is accountable, and is geared towards fairness, along with being easy to explain to the common man on how it functions. Excerpts:
When enterprises talk about AI, what are the different stages of AI? Is it only about creating algorithms and running data through them? What is the larger picture?
What do enterprises mean by AI? In a bird’s eye view, we can break AI into three parts. One is the data lifecycle, which refers to how you collect, clean and transform data. The second part is how to make the best use of data through optimisation, running it through AI models. The third stage is how it can be deployed so that it can provide impact. With deployment also comes constant learning.

How can you ensure that the right data is used for the AI models?
Many studies in Forbes and MIT show that a considerable amount of time for AI is spent in preparing data. There is currently no systematic effort in preparing data for AI. Most data scientists are engaged in their own siloed work, and there is a need for a systematic effort. How do we tell data scientists that this data is good for AI, or if it is not, what are the issues with the data and can some of them be remediated? These are the areas to focus on.
In terms of AI, What is data labelling? Why is it important?

Labels are descriptions allocated to data, using which AI models can categorise the data. But what if the labels are wrong? These labels are given through human annotation or another business process. In such a case, it doesn’t matter how good the AI model is, but it is only going to learn the wrong thing through wrong labelling. Similarly, the data could be biased. For example, for banking, historical data will show that we have given out bad loans, but the new AI models do not need to learn that data. The place to correct this is on the data side through better data quality.
What can the CIO do to mitigate data bias? Or does it begin at the level of AI research? The likes of which IBM Research engages in?
As researchers, our responsibility is to build the tools that the developers and researchers can use to detect if their data model is biased or not. What action they take is onto them. The tools are aimed towards fairness and explainability, and they have to be made available to everyone. IBM research has built five toolkits that have been made open-source and can be used by everybody. While we provide the tools, the real action lies in the hands of CIOs and other business/government heads.

A March 2022 study by IDC said that 60% of Indian enterprises will use AI for decision making by 2026, keeping this in mind, how crucial is it to ensure that AI bias doesn’t take place?
Bias, along with explainability, is the cornerstone of good decision making. Trust in AI is one of the biggest impediments to deploying AI at scale. Trust needs to be built by design into the models. Just like all the AI scientists look at accuracy, scalability and performance as the core metrics to optimise models, they also need to start looking at fairness metrics and trust metrics as initial starting points.
AI talent shortage is real, what is missing in the lifecycle of talent creation currently? Is something being missed out on?

We have been feeling the shortage of AI talent. More than the shortage of AI talent, we need to train our students slightly differently. The three parts of AI are data, learning and deployment. Most of our talent and effort is in the middle path. Most of our graduate and undergraduate students know how to build AI models.
We don’t teach the entire data lifecycle as a module, because in real-life the data and datasets won’t be readily available. Many times, the task involves collecting data, getting it labelled and cleaned. Students don’t realise that there are so many complexities to getting the right data. Focus on data needs to be much more rigorous.
The deployment is also a crucial element that needs focus. We need to inculcate how to scale, deploy, wrap it into APIs, how the feedback loop is enabled. It is not just software development or engineering; these are real technical challenges that might even need AI to solve.

What are some of the AI research projects that you have undertaken, with potential for large scale impact?
In terms of data quality, we are building algorithms to assess and improve data quality. We have been engaged in this research for a couple of years with critical customers and partners.
Testing AI models is also a huge area that needs work. For example, a model that is built to predict whether a person deserves a credit card. The developer says the model is ready to be deployed, but there has to be a governance officer, risk officer or an AI team who can certify or validate the AI model. We are building a toolkit to test AI models. It is similar to how software quality engineers test AI code, the key difference is that now we need to engage in focused testing.

For example, generating use cases just to verify the model on fairness. The developer now needs to create test cases that are realistic, but different from what the model has already been put through. Unless we automate this process, the developer will have the final call on how the model will work, without exhaustive testing, which is needed before a model is put into production.