
How graph technologies can help platforms detect fraud
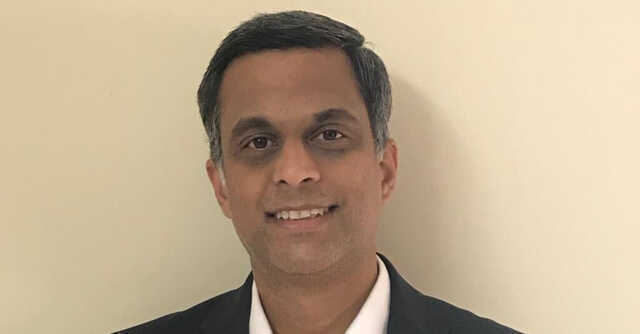

The pandemic accelerated digital transformation. Businesses, irrespective of their nature and industry, turned to digital solutions to sustain operations, build resiliency and even scale in some instances. India with our large base of internet users was no exception.
According to a report by Bain and Company, India’s e-retail market reached $38 billion in FY21. An EY-FICCI report suggests that OTT players in India gained 29 million paid subscribers in 2020. The Indian online gaming sector touched US$1.027 billion in 2020, states an All India Gaming Federation report.
Not just this, the pandemic also changed the way we work. Employees started working remotely and businesses adopted cloud-based solutions among multiple other changes to keep up with the changing demands and business environment. While this opened up doors to multiple new opportunities, it also provided a whole new playing field for fraudsters and cyber criminals.

According to RBI data, India’s digital commerce transactions grew 30% in 2021. While the country has been ahead of its peers in digital transactions since 2018, it is also witnessing a sharp rise in digital frauds. A total of 4,071 fraud cases were reported by Indian lenders in the first half of fiscal year 2021-22 and the amount involved in these frauds was Rs 36,342 crore. India lost at least Rs 100 crore every day to bank fraud or scams over the past seven years!
These numbers are alarming and as a result, security against fraud has become a prime concern for Indian organizations. Everyone wants to ensure that they have a robust framework that identifies threats beforehand and also helps combat attacks efficiently. While this happens, fraud still continues to cost them millions of dollars. A reason behind this is that traditional fraud detection methods and their analysis cannot keep up with the latest sophisticated threat actors who are now constructing evolved techniques to exploit flaws in the analysis systems.
Attackers today resort to financial and identity theft and can figure out if the user has fraud detection practices in place or not. If there are fraud detectors that operate manually, there will always be limitations of human capacity. As a result of these things, businesses are prioritizing security and looking for human machine teaming - for solutions that can strengthen their existing teams. Analysts want a solution that can help them focus on the critical scenarios and not make them skim through a large number of data points.

How do graph data platforms address this?
With business processes becoming increasingly automated, time margins for detecting fraud are reducing, increasing the need for real-time solutions. This is where traditional technologies lag since they are not designed to detect elaborate fraud rings. Many of the traditional fraud technologies built on relational platforms rely on set patterns. With fraudsters becoming more innovative and attacking through multiple directions by creating newer rings, it is imperative that the connectedness in the transactional data is studied.
This is where graph technologies come in. Graph data platforms are able to store relations and connections natively along with the data. It becomes much easier to detect innovative patterns in real time by observing traversals through the same nodes which would generally go undetected in traditional systems if this isn't a precondition that is set beforehand. In a nutshell, a graph helps express data. It collects all the data into one central location and stores nodes and relationships instead of tables, or documents.

Graph databases navigate deep hierarchies, find hidden connections between distant items, and discover inter-relationships between items. A graph data platform framework fights fraud by allowing one to detect and predict fraud and anomalies at scale, using the existing connections between the data points. It can identify patterns and create a feedback loop of insights. Graph technology uncovers a variety of important fraud patterns – all in real time – from fraud rings and collusive groups, to educated criminals operating on their own. It offers a variety of analytical approaches to identify fraud and anomalies, ranging from localized query patterns to machine learning–based insights.
To share an example, compliance expert KERBEROS needed a solution to check and document suspected cases of money laundering and, if necessary, report them to the authorities. They implemented a graph database solution that today maps approximately 150,000 people, companies and documents, as well as approximately 750,000 relationships between these entities. If suspicious transactions are detected, they are analyzed together with all relevant information and documents in the graph. Instead of taking a superficial look at relationships, legal experts can now uncover relationships only apparent at the second or third level.
An important tool of graph technology is the graph-optimized query language, Cypher, that guides the discovery of data patterns. A fraud analyst can flag people transacting with a known fraudster, or people two or three steps away from a known fraudster. Identifying people and nodes of interest is simple and efficient with Cypher. For similarity and community detection, the graph data platform provides out-of-the-box graph algorithms. On larger datasets where it's difficult to know exactly what you're looking for, these algorithms come in handy. These methods are frequently used to identify potential fraudsters and discrepancies that would otherwise go undetected if queries were used alone.

Together, these techniques lead not only to higher detection rates for fraud and anomalies, but also to higher accuracy, reducing the rate of false positives that require manual review or customer intervention. Graphs can therefore be effective to track the interconnected nature of fraud, crime, and anomalies. By leveraging connections to detect the undetectable, graph algorithms and graph machine learning enable organizations to stay ahead of new forms of fraud and crime.
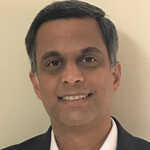
Rahul Tenglikar
Rahul Tenglikar is the regional director, India for Neo4j.