
Google’s AI-training supercomputers twice as power efficient as Nvidia’s
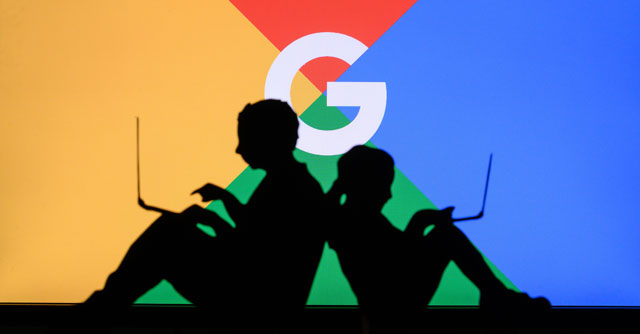

A team of Google researchers said on Tuesday that the company’s supercomputers, used to train a wide array of artificial intelligence (AI) algorithms and large language models, are nearly twice as power efficient as their industry counterparts. The research paper was submitted on April 4, and will be published at International Symposium on Computer Architecture (ISCA) 2023 — in June this year.
The research paper detailed Google’s latest generation custom chips used in its supercomputers, called the Tensor Processing Unit (TPU). The latter is the third supercomputer framework that Google is using to train and build its custom machine learning algorithms and tools, and this is further combined with custom ‘switches’. The latter are mediums that are used to connect multiple chips into a relay configuration — the fourth-gen TPU supercomputer at Google uses 4,096 chips, for instance.
According to the research paper, the combination of the custom processors and custom optical circuit switches have helped the company make its system almost 1.9x more power efficient than its cross-industry rival, the Nvidia A100-powered supercomputers. The new generation supercomputers have been in use since 2020, and are 3.1x more powerful than their predecessors, the TPU v3 supercomputer.

Google further said that the overall TPU v4 supercomputer is 10x faster than TPU v3, and its vast relay configuration has helped the company train its Pathways Language Model (PaLM) — the underlying language model behind its Bard chatbot. Other key metrics shared in the research paper cite 3x lesser energy consumption and 20x lesser carbon dioxide emissions in comparison with similar rivalling AI-training supercomputer architecture. However, Google didn't specify which industry rival it referred to in its energy metrics.
Large language models (LLMs), to be sure, combine billions of data points in order to be able to understand inputs in plain text, images or even sound, and offer human-like responses. Google’s rival firm, OpenAI, for instance, used 175 billion data points in the training dataset of Generative Pre-trained Transformer (GPT)-3.5, the LLM behind the ChatGPT chatbot. While there is no confirmation on the matter, GPT-4, the latest generation LLM used by OpenAI, reportedly uses over 3 trillion data points.
Companies around the world have projected that while big tech firms such as Google itself will have the means to create and train LLMs owing to the vast resources that they would consume, smaller firms around the world could take alternate approaches — such as customising a pre-trained LLM by plugging it into a custom dataset — to create various use cases of generative AI.
