AI & Data Analytics with Ashish Shekhar
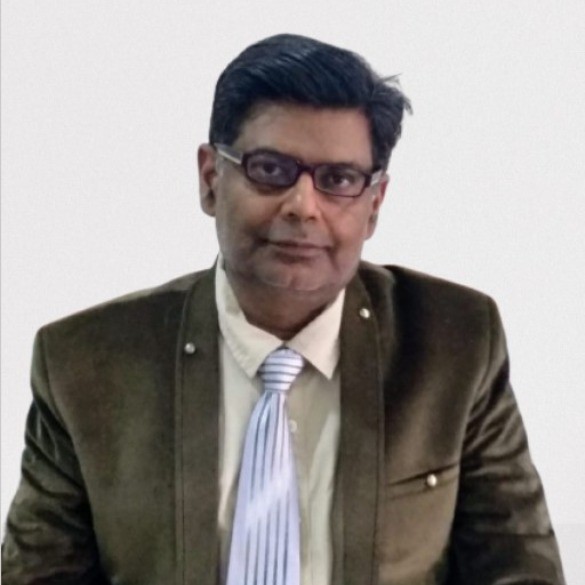
Question: Manufacturing environments often generate vast amounts of data from diverse sources (machines, sensors, etc.). How can manufacturers overcome challenges associated with data integration, ensuring a unified data foundation for effective AI implementation?
Ans- Data Integration and then Storing the data for utilisation, This process can be seen as a level 2 activity. However, many organisations are still struggling with phase 1, which involves generating quality data. The primary requirement is to initially produce high-quality data. Once this quality data is generated, the next step is to integrate it and then store it, marking the third stage.
The foundation begins with capturing the right type of data. According to AI principles, anything that can be captured and has recorded transactions can later be analysed for predictive analytics and other valuable purposes. While terms like IoT are commonly discussed, adoption is still limited, particularly among small and medium-sized industries. Recognising that data collection extends beyond manufacturing finished products is crucial. It involves collecting the right amount of high-quality data and having access to it, which is paramount.
Defining the work involves identifying the correct sensors to use and specifying the types of data to track, integrate, and store in tools like Azure Data Lake. Data quality is equally important, ensuring that data is seamless and standardised in format across locations. Inconsistencies in format can lead to significant issues, highlighting the necessity of standardising data formats.
Once these foundational aspects are addressed, the rest becomes more manageable. Various standard tools, such as Informatica for integration, can handle different types of data, including those that may initially seem unclear in terms of utility. By following this step-by-step process, organizations can lay a solid groundwork for effective AI implementation.
Question: While automation offers significant benefits, there's concern about workforce displacement. How can manufacturers leverage automation and analytics to create new opportunities and upskill their workforce for the future of manufacturing?
Ans- This is a significant change management issue for me. Organizations must acknowledge that the landscape has shifted. Just as people had to become tech-savvy with computers over time, now everyone needs to embrace data and analytics. This shift is crucial as decisions are increasingly data-driven across all sectors. Data analytics has become integral to virtually every job, from government institutions to financial sectors and supply chains.
Regarding automation, it primarily deals with streamlining routine tasks. However, by mastering data analytics, individuals can move beyond routine assignments and make data-driven decisions that automation might struggle with. This strategic shift not only reduces the risk of displacement but also enhances job roles to add more value. It's vital to foster a culture of continuous learning and adaptation, preparing the workforce for the future of manufacturing.
In conclusion, organizations must prioritize change management and rigorous training to equip their workforce with the necessary skills. Denial about automation's impact will only delay necessary actions, potentially leading to challenges down the line. Embracing data analytics and automation proactively positions the workforce to leverage these technologies for mutual benefit and future success in manufacturing.
Example:
In IT, there are two key terminologies: OLAP and OLTP. OLTP stands for online transaction processing, which was a buzzword about 15 years ago. Being able to record all transactions in enterprise software like SAP or Oracle was considered a significant achievement back then, ensuring seamless operations. However, merely having these systems in place is now considered a baseline requirement. The focus has shifted towards utilising this data effectively for decision-making and predictive analytics, a concept that was not prevalent a decade ago.
The transition to OLAP, or online analytics, is evident in the evolution of software like SAP to platforms like HANA, alongside the emergence of various third-party tools. This shift underscores the current importance of not just recording transactions but also leveraging data for insights and predictions. Staying on this trajectory means continuous evolution, both for the technology and the users, as the landscape of IT and analytics continues to evolve.
Question - Your experience spans manufacturing, IT project management, and supply chain management. Can you share a specific example from your career where you implemented a data-driven solution (AI, automation, or analytics) to address a major challenge in one of these areas? How did you measure the success of this initiative?
Ans- So I'll give you an Example on AI plus analytics, Purely Supply Chain management.
In a dynamic manufacturing environment with daily procurement challenges, traditional methods often fell short in efficiently managing vendor selection. Leveraging AI and analytics, we transformed this process by developing an algorithm that rated vendors based on their past performance metrics stored in SAP HANA. This automated system categorized vendors into four ratings, enabling quick and informed decision-making for procurement officers. By focusing on vendor lead times and performance rather than just price, we achieved a 20% reduction in procurement costs and lead times, significantly improving supply chain efficiency.
This data-driven solution not only streamlined vendor selection but also showcased the power of AI in enhancing decision-making beyond manual rule-based systems. The success of this initiative was measured through tangible metrics such as cost reduction and improved lead times, affirming the value of AI-driven analytics in modern supply chain management. The algorithm's success is further validated by its availability on Azure Marketplace, offering a scalable solution for businesses facing similar procurement challenges.
Question - There's growing interest in the potential of AI to contribute to sustainability efforts. What are your thoughts on this emerging trend? Can you share some examples of how AI can be used to optimise resource use or minimise environmental impact within the manufacturing sector?
Ans- AI has substantial potential to contribute to sustainability efforts in various ways within the manufacturing sector. For instance, computer vision technology can enhance waste management by quickly identifying defective products, reducing waste generation. Additionally, accurate demand forecasting through AI can minimize overproduction, preventing unnecessary waste and subsequent environmental impact.
Furthermore, AI-driven analytics and IoT sensors can optimize energy consumption, control emissions, and improve transportation efficiency. By automatically managing temperatures, optimizing routes, and reducing transportation time, companies can significantly reduce their environmental footprint and operational costs while enhancing sustainability practices.
*This is part of a community building initiative. The content is not produced by the editorial team and no Techcircle reporter was involved in its creation or publication.
“According to AI principles, anything that can be captured and has recorded transactions can later be analysed for predictive analytics and other valuable purposes.”
Ashish Shekhar, Group CIO, Makson Group of Companies