
AI transparency and interpretability will drive the next wave of automation in BFSI, says Arya.AI CEO
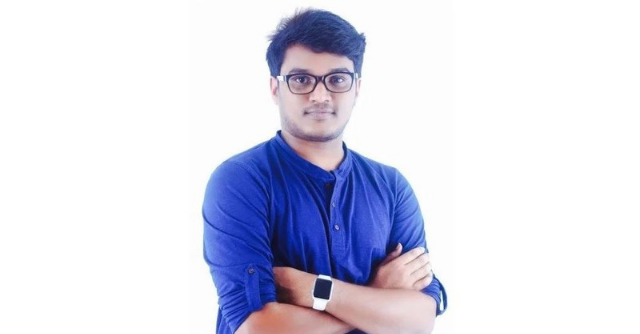

As the banking, financial services and insurance (BFSI) sector grapples with the challenges of adopting Artificial Intelligence (AI), Arya.AI, a company founded in 2013, is tackling the complex issues of AI interpretability and scalability. Originally focused on making AI accessible for enterprises, Arya.AI now concentrating on addressing the growing need for AI models that are transparent, aligned, and compliant with regulatory standards.
In a conversation with TechCircle, Vinay Kumar, Founder & CEO of Arya.AI, discusses the company’s evolution and the future of AI in BFSI. Kumar outlines how their solutions are helping financial institutions navigate the challenges of AI adoption, from automating routine tasks to tackling more complex problems that require long-term planning and coordination across multiple models. Edited Excerpts:
What core problems did you set out to solve when you started Arya.ai, and how has that focus evolved, especially with BFSI clients in India today?
When we started in 2013, there were no references, frameworks, models, or AI tools available. Our goal was to democratise AI, to help enterprises build and deploy AI quickly.

Today, the focus has shifted. Now we aim to make AI interpretable, aligned, and acceptable to all stakeholders so it can scale effectively. As model development becomes easier and more widespread, the surrounding layers, governance, interpretability, and risk, become critical.
Take large language models (LLMs) and foundation models. Just a few years ago, there were around 50 teams building them. Now there are over a thousand. But even with this growth, industries like financial services face challenges. These models are often black boxes, making them hard to trust, manage, or scale safely.
Users need visibility into how models work. They need to manage risk, ensure security, and meet regulatory standards. In India, we're still in the experimentation phase for frontier AI, focusing on internal or low-risk use cases with a human in the loop.

Last August, the Reserve Bank of India (RBI) released model risk management guidelines. These focus on traditional models, not frontier ones. But they signal that India is moving toward a more mature approach to AI adoption and governance.
This is where we come in. Most AI players in India offer models or solutions as a service. Very few focus on AI interpretability and alignment. We position ourselves as one of the few frontier labs not just in India, but globally addressing these core challenges.
As the Indian market matures, we aim to be the platform of choice for enterprises looking to scale AI responsibly and effectively.
So among your AryaAPI, Libra and AryaXAI, which area do you see as the future growth engine and why?

We’re approaching this with a dual focus, applications and platform. On the application side, there’s immediate value. Our parent company operates in the enterprise software space, and this is where we’re unlocking new potential. Traditional processes, like the standard 10-slide, 14-step workflows—are being disrupted by more capable AI agents that can handle complex tasks. We’ve partnered with Aurionpro Solutions and our customers to build and rapidly productise new applications.
On the platform side, through AryaXAI, we’re tackling deeper industry problems, things like interpretability and alignment. These are long-term challenges. Few organisations are focused here. For example, Anthropic recently invested $50M in mechanistic interpretability, and OpenAI used to have a team dedicated to it. We believe solving these issues now will enable us to build a mature platform ready for future, more advanced models like AGI. This is where our AI alignment labs in Paris and Mumbai come in.
So we’ve split the team, one group is focused on short-term gains through application innovation, while the other is working on foundational platform technologies for the long term.
How are your models fine-tuned to address BFSI challenges like fraud, underwriting, and credit scoring?

We offer two main products on the application side: Arya APEX (also referred to as Arya APIs) and Libra (also known as Autonomous Finance). Arya APEX is a suite of pre-trained deep learning models designed for specific use cases in the banking, financial services, and insurance (BFSI) sector. These APIs automate tasks such as signature matching on checks, forms, or account change requests, enabling automatic validation. They also handle document forgery detection by identifying tampering in KYC forms, bills, and other documents. In addition, Arya APEX includes capabilities like bank statement analysis and cash flow prediction by extracting and processing data from various financial documents. These APIs are built for plug-and-play use, focusing on data extraction, validation, and consolidation.
Libra, or Autonomous Finance, offers task-specific solutions powered by state-of-the-art neural networks. These models are fine-tuned using the customer’s data to support decision-making processes such as autonomous underwriting, fraud monitoring, and function optimisation. For example, Libra can automate the entire claims process for insurers or make underwriting decisions for financial institutions in areas like retail lending, trade finance, and guarantees. While Arya APEX focuses on delivering pre-trained APIs for data-related tasks, Libra is built for more complex, fine-tuned applications that require continuous model adaptation and decision automation.
What’s been the biggest challenge in making deep learning models explainable for BFSI clients?
We faced this problem around four or five years ago. That’s both the benefit and challenge of being early adopters of deep learning. We deployed our first deep learning model in 2016 for claims automation, and later for underwriting.

We hit a roadblock while productionising the underwriting product. The key issue was interpretability, underwriting can't be a black box. You need to explain decisions to risk managers or regulators, whether you approve or deny an application. Without that, the platform’s usefulness is limited.
The challenge was compounded by the use of deep learning. At that time, the models were smaller and task-specific, typically around 10 to 25 million parameters, sometimes up to 100 million with pre-trained models. Still, we needed interpretability. Existing open-source tools like SHAP weren’t reliable or defensible, especially in regulatory or legal discussions.
So we built our own solution. In 2021, we introduced and patented a technique called Deep Learning Backtrace (DLB) to interpret neural network behavior. That was our response to the interpretability gap.

Since then, model sizes and complexity have increased significantly, especially with the rise of LLMs. Fortunately, the DLB technique scales to LLMs too. We recently released a library based on it that can explain what’s happening inside any model, regardless of size or modality.
This is just the beginning. As models grow in complexity, understanding them becomes even more critical. Earlier, model training and inference were relatively manageable. Now, with huge models and advanced architectures, the black-box nature of these systems is more pronounced.
We’re now working on accelerating explainability to match inference speed. We're also exploring how to use interpretability for better model alignment. While the core technique remains the same, we’ve refined the implementation for scalability and use in newer contexts.
Looking ahead, we believe interpretability won't just explain decisions—it will drive alignment. That’s our focus for the next few years. Others in the space, like Anthropic, are also moving in this direction. By understanding how models work, we can better control risks, behaviors, and even hallucinations.
We're still in the early stages, but the potential over the next four to five years is significant, especially in mechanistic interpretability and model alignment.
Do you think the BFSI sector will move towards using larger or pre-trained models with the rise of generative AI and foundation models, or will specialised models continue to dominate?
Right now, the distinction between a Small Language Model (SLM) and a Large Language Model (LLM) mainly comes down to size and scope, SLMs are often tailored for specific industries. But the core challenges remain the same.
If a model isn’t interpretable, it can’t be trusted. If it can’t be managed from a risk perspective, it’s not usable. For example, in underwriting, where Non-Performing Assets (NPAs) range from 1% to 12%, even a 10% prediction error makes the model too risky for production—no matter how well it performs elsewhere.
So, the fundamental issues, interpretability, risk management, and compliance, must be addressed. Even if we had AGI tomorrow, it wouldn’t be useful without solving these problems.
From a regulatory standpoint, there's increasing focus on explainability. Accuracy alone isn’t enough to choose a model. A model might be highly accurate but still biased or unsafe in certain scenarios. Without understanding these risks, deploying such models is a blind gamble with societal impact. That’s why regulators are stepping in.
Ultimately, this isn’t about whether a model is an SLM, LLM, or AGI. It’s about whether the model is interpretable, manageable, and compliant. These factors determine whether banks or other institutions will use them in sensitive, mission-critical settings.
You could have AGI in six months, but without addressing these issues, it won’t be used beyond low-risk applications like chatbots or assistants.
Your company recently announced the launch of AI alignment labs in Paris and Mumbai. How will these labs help develop scalable frameworks for AI explainability and alignment?
We’re focused on solving two core challenges: interpretability and alignment. We believe these are connected, so we’ve heavily invested in interpretability and are now expanding our work into alignment. Our goal is to lead in both areas.
This is the vision behind setting up our labs in India and Paris. In Paris, we benefit from strong foundational research talent and government support, through grants, tax credits, and other programs across France and the EU, which makes it an ideal place for an R&D lab focused on these problems.
In India, while application-level innovation is strong, there’s a gap in foundational research. I've been highlighting this for years, and now we're investing in building that ecosystem. Our aim is to help India contribute to foundational AI research that could support future global innovation. This is a long-term commitment we're funding independently.
The labs will work on new techniques for interpretability, develop open-source tools, like the Deep Learning Backtrace Lab (DLB) and XAI Evals—and release more in the future. We’re committed to making much of our research open source.
Finally, talent is key. The most impactful AI companies are built by people solving hard problems. This lab is meant to attract and develop that kind of talent, especially in India, where we want to go beyond application work and tackle foundational AI challenges.
How do you see generative AI models impacting automation and decision-making in the BFSI sector over the next three to five years?
Current large models and AI agents are effective at handling short, straightforward tasks that require limited planning, typically three to four steps. However, enterprise problems tend to be broader and more complex. These often still require human experts, especially for handling exceptions. Extending AI capabilities to these scenarios is the next major goal for LLMs and other advanced systems.
Relying solely on language models isn't enough. Multimodal systems are emerging, including models for speech, vision, and tabular data. Model scale appears to play a key role, larger models tend to solve a wider range of problems.
On the agentic side, there's growing investment in long-term planning. One example is deep research, where models are given more compute time to reason before generating an output. This supports use cases that involve multiple tools and models working together, like underwriting for large businesses or detecting fraud in complex financial transactions.
In such cases, no single model is sufficient. Solving these problems will require a combination of models and tools operating in coordination.
We expect rapid progress in this area over the next one to two years. Agentic frameworks will improve in planning and problem-solving using a mix of models and tools. Within three to five years, enterprises could see the deployment of specialised AGI-like systems internally, even if general AGI remains out of reach in the public domain. This shift could mark a major transformation, moving from traditional workflows to systems driven by autonomous agents.