
AI inferencing: The real-world engine driving the next wave of enterprise innovation
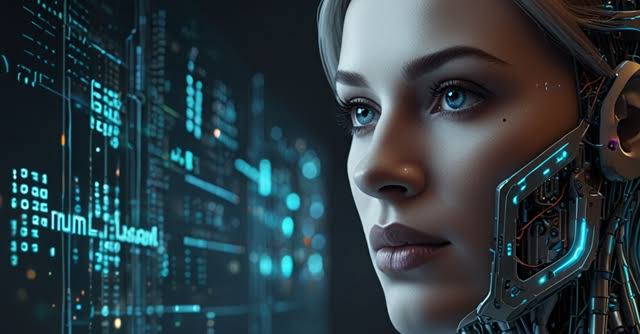

In the world of Artificial Intelligence (AI), the spotlight often shines on large-scale model training with massive datasets and billions of parameters. However, the real test of an AI model is not limited to how it is trained; it also involves how it performs in the real world. This is where AI inference steps in.
AI inferencing is a process where a trained AI model applies its learned knowledge to solve a task or generate an output. In the case of AI inferencing, a model is capable of making predictions on previously unseen data. From fraud alerts that trigger in milliseconds to real-time language translation on the phone, inferencing is quickly becoming the next great frontier in AI.
It offers compelling advantages such as real-time decision-making, personalised user experiences, and improved operational efficiency. Unlike training, inference is faster, more scalable, and can be deployed cost-effectively, often at the edge, closer to users. Today, inference powers everything from medical imaging diagnosis in healthcare to fraud detection and chatbots in finance, to smart recommendations in e-commerce, and navigation in autonomous vehicles.

Referring to a recent video of an autorickshaw driver conversing in Kannada and a customer conversing in Hindi that went viral for the use of an AI Voice Assistant using ChatGPT, Ranganath Sadasiva, Chief Technology Officer, HPE India, said: “This is an easy and innovative example of how AI inference can be useful.”
He added that while this is one of the many use cases for AI inferencing, there are thousands of opportunities across the spectrum of industries enabling real-time analysis, predictions, and decision-making.
Digital experience platform Contentstack is using AI inferencing to integrate intelligence in every layer — from smart authoring tools to automated tagging and dynamic personalisation. “While we invest in foundational AI capabilities, inference is how we make AI useful for our customers,” said Suryanarayan Ramamurthy, head of data science.

As per market research firm Markets and Markets, the global AI inference market is set to grow from $106 billion in 2025 to $255 billion by 2030, growing at a CAGR of 19.2% for the period.
Cost-effective alternative
Companies are racing to achieve high-performance AI inferencing because it's where AI creates real-time, tangible value for business operations and customer experiences. It is also reflective of the change in the generative AI market, where AI use cases are expanding to the enterprise.
Nvidia, which has been the undisputed leader when it comes to generative AI compute infrastructure, is feeling a lot of heat when it comes to taming the bull that is AI inferencing.

Nvidia's GPUs, optimised for high-performance training tasks, often consume significant power during inference operations. This can lead to higher operational costs, especially when deploying models at scale.
Competitors like AMD and startups such as Cerebras and SambaNova are introducing inference-focused chips that promise better performance-per-watt ratios, appealing to enterprises seeking cost-effective solutions.
In an earlier interview with TechCircle, data center company NxtGen Technologies’ CEO AS Rajgopal said that Nvidia holds a dominant position in the generative AI chip market, making it ‘near-impossible’ for price negotiations. “The reliance on high-cost GPUs can be reduced by building smaller, optimised models. This is the key advantage of inference, which allows AI to operate without the heavy compute demands of training.”

NxtGen, along with Nvidia and AMD, has also partnered with Santa Clara-based d-Matrix that specialises in inference-specific GPUs, offering a more cost-effective alternative.
Closer home, IIT Madras-incubated deeptech startup Ziroh Labs created a lot of buzz earlier this year when it demonstrated a CPU-based AI platform that is capable of running large AI models without the need for expensive GPUs. Called Kompact AI, it has been optimised for models like DeepSeek, Qwen, and Llama, demonstrating efficient performance on standard CPU hardware. With this approach, Ziroh Labs claims that it has been able to cut down inferencing costs by 50%.
“India is a developer economy; it comprises many programmers and developers. And developers want to build applications. They don’t want to (and don’t need to) build models from scratch. They want to plug into existing models and focus on inferencing.

That’s why having the right inference infrastructure in India is critical. We need to empower developers with the right tools and the ability to run inference across various devices. That’s exactly what we’re solving for. And when you think about it, most households today already have at least five CPUs. So if there’s a developer in the house, they don’t need to wait anymore—they can start building AI-powered applications right away,” Hrishikesh Dewan, Ziroh Labs CEO, told TechCircle.
Challenges persist
AI inferencing, while promising, also poses several challenges. One of the more persistent challenges is that of latency. Inference requires fast, low-latency predictions, especially in real-time applications like fraud detection or autonomous driving. Achieving this speed, particularly at scale, often demands expensive hardware, which increases operational costs.
A possible solution to this problem is deploying inferencing models on the edge. However, for large models, computing power becomes a constraint. Moreover, to make models suitable for inference, especially on lower-end devices, they often need to be compressed or quantised. This can lead to accuracy degradation if not done carefully.

These challenges have led designers to work on possible solutions.
“As businesses adopt AI, the focus is shifting to making inferencing more cost-effective and efficient. High GPU costs, limited availability, and energy demands make large-scale deployment challenging,” according to Ganesh Gopalan, CEO of Gnani.ai, a voice AI company.
“Companies are exploring alternatives like edge computing, CPUs, and optimised GPU usage to improve ROI. On the software side, techniques like intelligent caching and reducing token usage help lower latency and improve margins. The goal is to make AI deployment scalable, fast, and financially viable,” he added.
AI inferencing is fast becoming the engine of real-world AI adoption, driving tangible value across industries. As cost, latency, and scalability challenges are addressed, it will define the next wave of enterprise innovation.