
Genesys exec on how AI and precision geospatial data are redefining urban planning and beyond
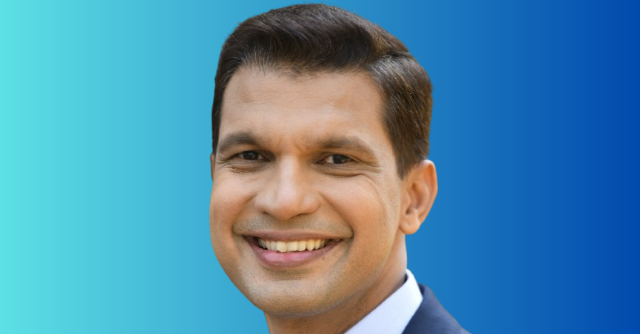

Geospatial technology has advanced significantly in the past two decades, improving urban planning, infrastructure, and environmental management through precise data and Artificial Intelligence (AI).
Genesys International Corporation has been working in this field for nearly 20 years, creating detailed 3D digital models of cities and integrating diverse data sources to support projects like flood management, electric vehicle routing, and navigation.
In a conversation with TechCircle, Sameer Sankhe, Chief Digital Officer at Genesys, discussed how the company uses unique data and AI to expand geospatial applications beyond mobility, into areas such as agriculture, disaster response, and climate resilience, and more! Edited Excerpts:
Genesys has been in the geospatial space for nearly two decades, what has driven your long-term vision, and how have you seen the sector evolve over the years?

We began by focusing on large-scale national problems and developed new tools to address them. One of our core innovations is the creation of India’s first 3D digital twin for urban areas. These are highly accurate 3D replicas of cities, built with five-centimeter precision.
One use case is infrastructure planning. For a proposed road project in Prabhadevi, we overlaid the planned development on the 3D model. This helped visualise the impact, including which structures and trees would be affected. The Brihanmumbai Municipal Corporation (BMC) is currently using this system for planning purposes.
The platform also supports sustainability analysis. It can perform shadow analysis based on time of day and year to estimate solar potential. For example, in Varanasi, we used this tool to calculate rooftop solar capacity for the entire city, considering building orientation, weather conditions, and shadows from nearby structures. The tool can also provide solar potential for individual homes.

Sensor data, such as CCTV feeds or air quality readings, can be integrated into the platform. It's interactive and supports additional use cases like identifying unpaid property taxes, which can significantly increase revenue for municipal corporations.
Our work is built on three core capabilities: 3D digital modeling, 2D mapping (similar to Google Maps), and street-level imaging. Together, these datasets help address challenges like flooding. We use digital elevation models to identify low-lying areas prone to flooding. During last July’s heavy rains, Pune municipal authorities used our data for preparedness and analysis.
The elevation data also supports electric vehicle (EV) routing. For example, traveling from Pune to Mumbai uses less charge than the reverse route due to elevation gain. Within cities, elevation data helps optimise routes that include flyovers and slopes, improving EV range predictions.
You recently launched an AI-powered navigation map with augmented reality and intelligent speed assistance. How do these features improve the driving experience?

When you use a map in a car, your first interaction is usually through search. Today, that process is clunky. You have to type in queries, which can lead to errors or misunderstandings. The system doesn't always recognise what you're trying to say.
To improve this, we built AI-powered navigation. It's not just for search, it supports exploration. The system taps into a large database of over 35 million Points of Interest (POIs), including schools, shops, hospitals, and fuel stations. This is one of the largest POI databases in the industry.
The AI can handle complex natural language voice commands. For example, if you say, "Take me to a seafood joint on the way," the system identifies seafood restaurants along your current route. To do this, the AI must be deeply integrated with the map to understand both routing and relevant POIs along the path.

We demonstrated this to a German automotive OEM in Bangalore. They tested it by asking how long it would take to reach the airport, and then followed up by asking when they should leave home to get there as fast as possible. The system accurately responded to both.
These models are trained on proprietary data: our maps, routes, and geographic insights. This makes the system different from general-purpose AI tools like ChatGPT. The automotive industry is showing strong interest in this kind of AI-driven functionality.
With AI-powered route planning and voice assistance, how do you see the future of in-car navigation evolving from an industry perspective?
Cars are now the third most-used space after home and office, making the in-car experience a priority. Modern cars are software-driven, and maps play a central role, not just for navigation, but also for EV range, ADAS, and autonomous systems.

Our high-precision maps (accurate to 5 cm) support immersive 3D environments, intuitive interfaces, and real-time updates. For EVs, our software calculates range using 20+ parameters and suggests optimal charging routes based on live data.
We’re also enabling in-car commerce, drivers can receive real-time offers from nearby vendors. For safety, we’ve developed ADAS-ready maps using our own "Genesys Constellation," which includes aircraft and vehicles equipped with LiDAR and cameras.
Through age-based incremental map updates, we plan to use ADAS camera data from cars on the road to keep maps current, helping avoid hazards like sudden lane changes or road closures.

Our HD maps capture over 200 attributes, including elevation, road curvature, and lane-level data. These support critical functions like speed control, lane guidance, and early warnings in autonomous and driver-assisted systems.
Your company has created digital twins for cities like Ayodhya, Varanasi, and the complex area of Dharavi, how do these digital replicas aid urban planning and infrastructure development, and what challenges did you face in mapping such a densely populated area?
Capturing data in dense urban environments like Dharavi poses unique challenges. The sensors used in flights, for example, are extremely expensive, often five times the cost of the aircraft itself. The backpack system is also costly, running into several crores. To handle such complexity, we sourced the best backpack LiDAR system from a US-based manufacturer.
When we told them we planned to map Dharavi, they said the densest area they had mapped was Venice. But Dharavi is significantly denser. In some places, buildings are just two feet apart, with very little light in between. Drones can’t accurately capture such environments. That’s why we chose the backpack.
Our engineering team modified the backpack specifically for use in Dharavi. These customisations allowed us to successfully map the area. After the deployment, the original equipment manufacturer (OEM) invited us to the US to explain how we managed to do it.
The mapping process starts with data capture, followed by processing. The raw data runs into petabytes, requiring high-performance software and proprietary algorithms we’ve developed to generate accurate 3D replicas, with up to five-centimeter precision.
This data enables several applications, such as shadow analysis, building measurements, and onboarding tools. These applications are part of an end-to-end workflow, from data capture to final delivery.
Because the dataset is so large and detailed, it requires both manual and automated processing. Over the years, we’ve developed email-based algorithms that speed up the work and improve accuracy.
Finally, we build applications based on this data. One example, already in the public domain, involves a developer currently using the data. This has been a technically demanding but socially important project for the city, and we’ve completed it successfully.
With rapid AI and machine learning advances, how are companies planning to stay ahead in geospatial technology? How do you see AI evolving beyond mobility, into areas like agriculture, disaster management, and climate resilience?
The impact of our services goes well beyond mobility. They are also relevant to areas like agriculture, climate, and land ownership.
AI and other digital technologies rely heavily on data. Without quality underlying data, AI has limited use. Genesys has access to unique datasets, which allows us to do things that aren't otherwise possible. We apply AI algorithms to our proprietary models to generate valuable insights.
For example, using our street-level and 3D measurement tools, we can capture and analyse vegetation. This capability supports various sustainability efforts. One state government asked us to map its forest areas. The resulting data provided detailed analytics on green cover and vegetation diversity by capturing spectral imagery.
In agriculture, similar imaging techniques help analyse crops and detect diseases. We also process this data to support farming decisions.
Our data is used in land mapping too. Land disputes are a major contributor to case backlogs in Indian courts, tying up a significant portion of GDP. Digitising land records can help address this issue.
In terms of climate impact, our data plays a role in flood management. It helps predict where floods are likely to occur. Our work has also supported disaster response. For instance, after the landslide in Joshimath near Kedarnath about 18 months ago, we created a highly accurate map of the area free of charge. This helped the government analyse both the causes and impacts of the event.
These examples show how our work contributes to rural development, agriculture, environmental monitoring, and land management—extending well beyond urban planning and mobility. Our dataset exceeds 10 gigabytes, making it a valuable foundation for applying AI at scale across multiple sectors.