
Working with startups, big firms to build AI ecosystem: Nvidia India’s Vishal Dhupar
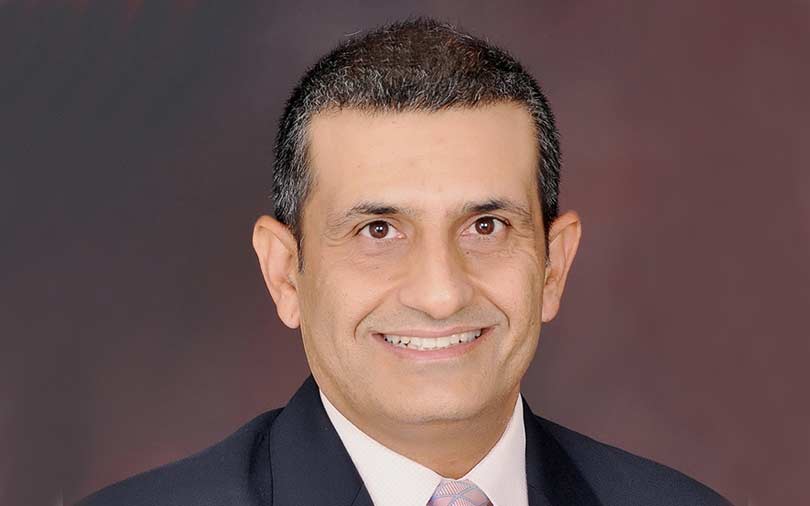

Nvidia Corp, best known for its graphics cards for personal computers, has been helping Indian developers expand their capabilities in artificial intelligence (AI) and deep learning (DL). The company conducts workshops on deep learning in six cities and provides training on the latest techniques for designing, training and deploying neural networks across numerous application domains. It helps startups and large corporations in India understand how GPU, or graphics processing unit, computing works. In a conversation with TechCircle, Vishal Dhupar, Nvidia’s India managing director, spoke about the firm’s proprietary technologies in AI and ML, the problems it is solving in deep learning and its plan for India. Excerpts:
Many companies, including Google, Microsoft and IBM, are talking about developing products and services around artificial intelligence and machine learning. Where does Nvidia fit into the picture?
Nvidia is at the core of most AI and ML solutions being developed today. The company, founded by Jensen Huang in 1993, began its journey as a visual computing company identifying a space in PC gaming. It was then that we started producing graphics cards and attaching them to PCs so that central processing units (CPUs) could handle the extra load of graphics processing.

But that showed us that these GPUs could be used to take care of heavy computational loads that other processors couldn’t within a desired timeframe. Thus, we came up with products such as Quadro, Cuda and Tesla that can offer different capacities when it comes to computing workloads.
Cuda is a parallel computing platform and application programming interface model. Quadro GPUs are designed for high-performance design workstations where industrial grade designing, advanced special effects and complex scientific visualisation are worked upon.
Tesla GPUs are used for the most demanding high-performance computing needs and run hyper-scale data centre workloads. Tesla products are often used by scientists and data researchers looking to scan through petabytes of data to create an AI model.

So, Nvidia GPUs power the computational workloads running on AI and deep learning platforms. These GPUs also help provide the end-user or the research organisation the ability to choose the basic framework of neural networks on which the platform will be deployed. The framework could come from multiple houses – TensorFlow from Google, CNTK from Microsoft or a few other open source ones from universities.
What is the advantage of having GPUs over traditional CPUs?
In typical neural networks, there are a million parameters which define the model and it requires large amounts of data to learn these parameters. This is a computationally intensive process, which takes a lot of time.

Typically, it takes days to train a deep neural network. By using GPUs, which can handle computational activity (distributed algorithms) in multiple cores running in parallel, we can speed up this process.
Imagine the amount of data that we are generating today and we will generate in the future. The neural networks need to use deep learning to keep learning from the data being gathered. Think of scientists who are trying to come out with a new drug. They have to train the AI engine on huge sets of data but they don’t have the time to wait their entire life cycle.
What other challenges is Nvidia helping solve for deep learning?

In deep learning, there are two action items: One is to develop the training modules so that the system can learn from the data over a period of time. This is a huge computational graph. The second is to deploy the AI at the end device such as a car, a thermometer or a camera.
However, each device has its own characteristics in terms of data acceptance rates or computational power. Engineers need to fold the computational graph for the devices as needed so that it suits synchronisation between the training module and the deployed module.
For this reason, Nvidia has created a framework to optimise the inference (data sent back to the neural network) called TensorRT. It is a programmable inferencing module [that determines whether] the end device can cater to its characteristics. We released TensorRT last year with the goal of accelerating deep learning inference for production deployment.

Why does ‘inference’ need a specific solution such as TensorRT?
As consumers of digital products and services, we interact with several AI-powered services every day such as speech recognition, language translation, image recognition and video caption generation, among others.
Behind the scenes, a neural network computes the results for each query. This step is often called ‘inference’, wherein new data is passed through a trained neural network to generate results. In traditional machine learning literature, it’s also sometimes referred to as ‘prediction’ or ‘scoring’.

This neural network usually runs within a web service in the cloud that takes in new requests from millions of users simultaneously, computes inference calculations for each request and serves the results back to users. To deliver a good user experience, all this has to happen under a small latency budget that includes network delay, neural network execution and other delays based on the production environment.
Similarly, if the AI application is running on a device such as an autonomous vehicle performing real-time collision avoidance or a drone making real-time path planning decisions, latency becomes critical for vehicle safety. Power efficiency is equally important since these vehicles may have to go days, weeks or months between recharging.
However, once the AI is trained and about to be deployed, current techniques or processes often fail to deliver on key inference requirements such as scalability to millions of users, ability to process multiple inputs simultaneously, or ability to deliver results quickly and with high power efficiency.
TensorRT can address these deployment challenges by optimising trained neural networks to generate deployment-ready inference engines that maximise GPU inference performance and power efficiency.
In what sectors is Nvidia working on AI?
We have announced partnerships to further AI in key vertical industries. These include initiatives with GE Health and Nuance in medical imaging, with GE company Baker Hughes in oil and gas, and with Japan’s Komatsu in construction and mining.
We have formed several partnerships in the automotive space after releasing AI self-driving platform DRIVE. We signed an agreement with ZF and Baidu to create the first production AI autonomous vehicle platform for China. We have partnered with Volkswagen and Mercedes-Benz to integrate AI into their vehicles.
We are also working with China’s Alibaba and Huawei to roll out their Metropolis Smart Cities platform. Our video platform Jetson has the ability to bring AI inside security cameras being deployed across major cities of China.
What companies are you working with in India?
We have the Inception programme running in India under which we were looking at companies that would need to run AI workloads. Our aim was to connect to these people to understand what kind of platforms they were using and to help them understand how GPU computing could solve their fundamental problems.
These companies, which include early startups to major corporations running research labs in India, are from the healthcare, language processing, educational systems, automotive and the engineering sectors.
The sole objective was to bring together the local ecosystem of AI developers, data scientists, researchers and academia to look at AI trends, technology, use cases and India’s role and opportunities in what many are calling the fourth Industrial Revolution. Use cases could range from tackling energy issues to predicting soil mixture for agricultural purposes.
We also have a Deep Learning Institute, which Nividia formed in 2016, to provide hands-on and online training in AI worldwide. It is already working with more than 20 partners, including Amazon Web Services, Coursera, Facebook, Hewlett Packard Enterprise, IBM, Microsoft and Udacity.