
Now, an AI engine that combats facial-recognition detectors crawling social media
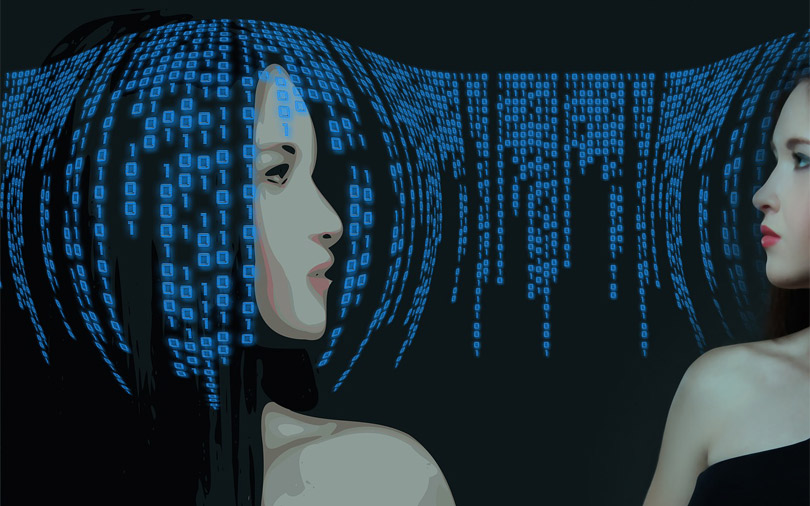

Researchers from the University of Toronto have come up with an artificial-intelligence technology that thwarts facial recognition on social media, thereby protecting your personal privacy, news portal ScienceDaily reported.
"Personal privacy is a real issue as facial recognition becomes better and better," professor Parham Aarabi, the lead researcher of the project, was quoted as saying. "This is one way in which beneficial anti-facial-recognition systems can combat that ability," said Aarabi, who is working on the project with graduate student Avishek Bose. The report said that the new filter has been developed using adversarial training -- a deep learning technique to impart human-like intelligence by pitting two artificial intelligence engines against each other -- one that identifies faces and the second that works to disrupt the facial recognition done by the first.
In the end, a digital filter came out, just like the ones you see in your smartphone camera app or Instagram, which can be applied to photos to protect privacy.

The report said that the usage of the filter allows changes in pixels of the picture that are not noticeable by the human eye. "The disruptive artificial intelligence can 'attack' what the neural net for the face detection is looking for," Bose was quoted as saying. "If the detection artificial intelligence is looking for the corner of the eyes, for example, it adjusts the corner of the eyes so they're less noticeable. It creates very subtle disturbances in the photo, but to the detector they're significant enough to fool the system," Bose said.
The researchers have tested the filter on something called a 300-W dataset, which is an industry standard for artificial-intelligence recognition technologies. Test results have shown that the filter can be used to scale down identification of faces from 100% to 0.5%.
"The key here was to train the two neural networks against each other -- with one creating an increasingly robust facial detection system, and the other creating an ever stronger tool to disable facial detection," Bose was quoted as saying.
