
MIT researchers create neural networks that imitate human reasoning skills
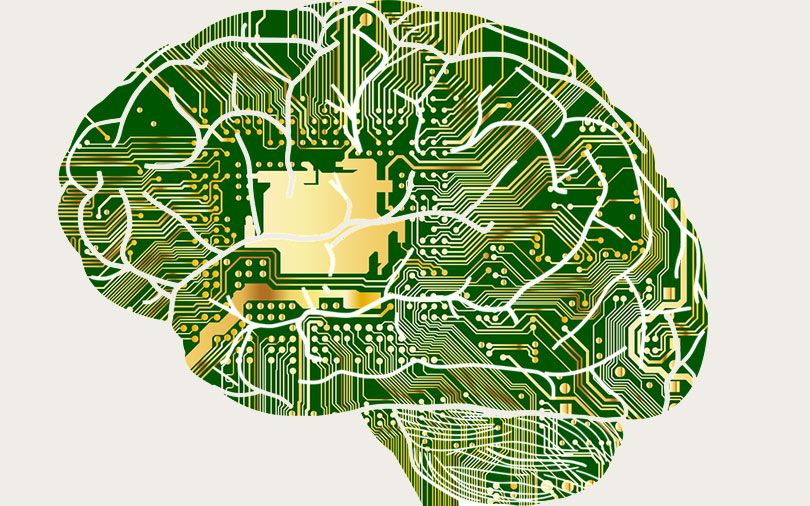

A team of researchers from the Massachusetts Institute of Technology has developed an artificial intelligence system that mimics human reasoning abilities, the institute said in a blog post.
The researchers, who work at MIT Lincoln Laboratory's Intelligence and Decision Technologies Group, created the system using a neural network that can answer questions about the contents of images, the blog post stated.
The model, named ‘Transparency by Design Network (TbD-net)’, converts thought processes into a visual format as it solves problems, allowing human analysts to interpret its decision-making process.

According to the blog, the model outperforms even the best visual-reasoning neural networks available at present.
This model has helped researchers clarify how neural networks arrive at decisions, which has long been a challenge. These networks, which simulate human brain functions, have become so complex that it is difficult to follow and understand their internal workings. “That's why they are referred to as ‘black box’ systems, with their exact goings-on inside opaque even to the engineers who build them,” the blog post stated.
With the new model, the developers want to make it clear how neural networks work in order to better interpret an AI's results, the blog post said.

For example, neural networks used in self-driving cars must distinguish between a stop sign and a pedestrian. The new TbD-net model provides researchers insights into how these networks come to make such differentiations, the blog post added.
"Progress on improving performance in visual reasoning has come at the cost of interpretability,” says Ryan Soklaski, who built TbD-net with fellow researchers Arjun Majumdar, David Mascharka, and Philip Tran.
With TbD-net, the MIT team was able to bridge the gap between performance and interpretation.

The researchers presented their work in a paper called ‘Transparency by Design: Closing the Gap Between Performance and Interpretability in Visual Reasoning,’ at the Conference on Computer Vision and Pattern Recognition (CVPR) this year.