
Column: Realizing real world benefits with artificial intelligence
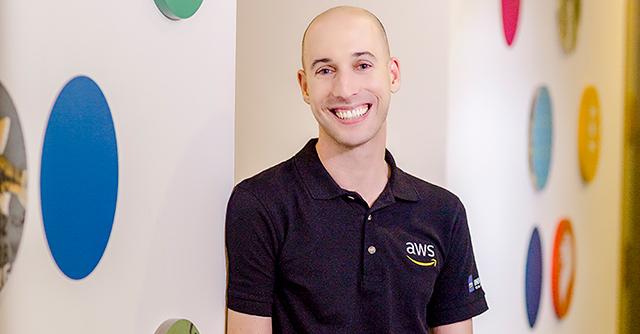

It doesn’t seem long ago that AI (artificial intelligence) and ML (machine learning) existed only in the imaginations of science fiction writers.
But in the past decade, we have seen huge leaps forward in computing power and the algorithms that use it, enabling machines to perform tasks that were previously only possible with humans.
Whether it is recognising content in pictures and videos or finding patterns of fraud in financial transactions, AI and ML are proving to often be faster and more reliable than humans, able to consume and analyse more data than any one person ever could. AI and ML are opening up possibilities for new products and services that would make any science fiction writer proud – including how we enjoy televised sports such as cricket!

Imagine a scenario where a batsman is considering taking a risky single. An AI system can easily calculate probable outcomes using many variables – such as the distance to be covered, the speed of the runner, and the likelihood of a fielder returning the ball and removing the bails – and then instantly make a judgement for viewers at home as to whether the batsman would have been safe or not.
We know such predictions are possible because we’ve already seen a customer apply this concept to the sport of baseball to predict the chances of runners stealing bases.
Major League Baseball created a deep neural network that predicts stolen base success using real-time and historical data, including the runner’s speed and burst, the catcher’s response time, the pitcher’s velocity and handedness, the lead-off distance, and the current game situation.

We are also seeing AI technology applied in Formula 1 racing. During each race, 120 sensors on race cars generate 1500 streaming data points every second, enabling Formula 1 to capture and process key performance metrics for each car during every twist and turn. By deploying advanced machine learning, Formula 1 can then pinpoint how a driver is performing and share those insights through graphics and visualizations on television broadcasts and digital platforms, giving fans access to the inner workings of their favourite teams.
The predictive power of AI and ML also has very practical applications in industry. Take for instance a factory where floor operators are keen to maintain their machines and avoid costly breakdowns. By placing acoustic sensors around the factory, it is possible for algorithms to detect auditory changes in vibrations and indicate whether or not a machine is performing optimally or about to fail.
Without AI and ML algorithms that can analyse and interpret the vast volumes of data being collected, such a system would be impossible to implement.

Importantly, while AI and ML technologies have become more powerful, they have also become more accessible. A vast range of tools are now available as off-the-shelf packaged services, ready to be trained up and used by organisations with minimal technical capabilities - and certainly without needing a cohort of data scientists and technicians.
Developers can now quickly and easily build, train, and deploy machine learning models, and apply them to real-world tasks. All developers need to do is provide data to train an algorithm, with minimal guidance to help it learn.
There are also AI systems that can be added to existing applications. These include services that can perform a variety of tasks, such as analysing images or video content to identify lost children in crowds or scan through video to flag inappropriate content, convert text to lifelike speech, or even make it easy for developers to create individualized recommendations for customers within their applications. Developers can also now automatically pull textual and unstructured data from large amounts of documents to derive relevant and meaningful insights from text.

And we are also putting even more complex tools into the hands of businesses and organizations, including a less commonly used form of ML called reinforcement learning.
Regular AI systems are trained by providing it examples to learn from, such as showing it a large number of images that contain birds to help it learn what a bird looks like. But in reinforcement learning, the AI system is given a task to perform, such as autonomously navigating a car around a racetrack. When the system makes the right decision, such as slowing down and turning appropriately when reaching a bend, it is rewarded. Hence, good behaviour is reinforced.
We brought this idea to life recently in the form of a robotic 1/18th scale race car that is trained to navigate on its own using reinforcement learning. It works by first creating a virtual car and racetrack environment in a computer’s memory. Then, a computer ‘driver’ pilots the car around the track repeatedly, constantly experimenting with how it reacts to the virtual view from the car’s cockpit. Instead of being told to speed up on a straightaway but decelerate before a turn, the virtual driver learns these behaviours by forming opinions about how to act, trying them out, and then grading itself on how well it did.

Simulating the racetrack environment enables the AI driver to be trained thousands of times, and the knowledge it gains in the virtual world is then used by a robotic car to navigate its way around a track in the real world.
By experimenting with reinforcement learning, we can create new applications for AI systems where regular methods would fail, such as training rescue robots to navigate through burning buildings where there are no reliable floorplans available.
These initiatives are helping customers understand that even the most sophisticated AI applications are now within their reach. But, the most exciting part is when we see these tools used in ways that even we have never considered, to solve real-world problems and do things that would never have been possible before.

Gabe Hollombe, is senior technical evangelist at AWS. The views expressed in this article are his own.
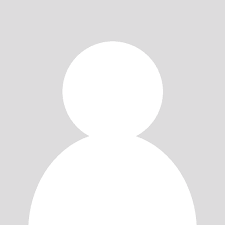