
Data security and privacy in the age of data-driven decisions
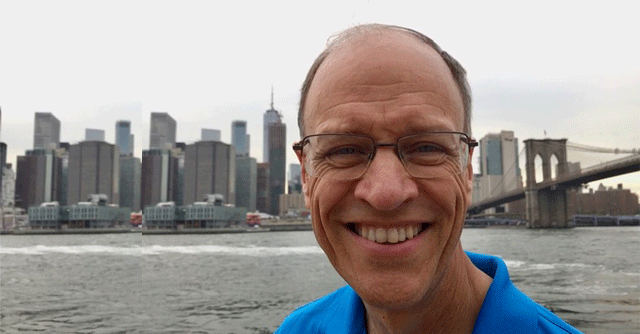

Companies are relying more heavily than ever before on technology to conduct business and to grow their customer base. In today’s hyperconnected digital era, consumer data is abundant and ubiquitous. Businesses have learned how to use it to predict consumer behaviour, so it has also become very valuable. All of this is well known to hackers, who are working overtime to gain access to this goldmine: we are now witnessing an unprecedented rise in data breaches. Technology also plays a role here, as cybercriminals rely on hacking software to make it easier to steal identities or gain financial access through stolen credit cards.
Consumers expect that the data they entrust companies with is protected and remains private. If this expectation is not met, they resent it very strongly, which explains why these recent, very publicized data breaches have significantly eroded consumer’s trust in businesses. At the same time, the costs of data breaches are rising year over year for businesses. Recent research from IBM Security tells us that lost business is the biggest contributor to data breach costs: the more customers lost, the higher the costs.
How can companies build in a strong compliance system to prevent and detect data breaches?
After several years of laissez-aller, we believe that companies are finally taking security and privacy much more seriously, heavily nudged by regulation such as GDPR and CCPA, which forces them to protect sensitive consumer data. With a much larger exposure surface and an evolving threat landscape, C-level executives are accepting more easily the reality that the cost of keeping sensitive data secure is increasing.

Security technology is part of the equation. Antivirus software, firewalls, intrusion detection systems, data encryption and single point of access with advanced, multifactor authentication are security measures that help with preventing breaches. But these won’t eliminate the risk of experiencing a breach: the IBM study data tells us that the odds of experiencing a breach are actually increasing. Systems are getting harder to secure because of their complexity; the mobile, IoT (internet of things) and cloud revolutions have greatly increased the exposure to new risk. In addition, every person that interacts with the corporate system can be a potential vulnerability: phishing attacks are often successful because it just takes one employee to click on a malicious link.
Therefore, companies need to bring both best practices and staff awareness training programs to the mix. On the best practices side, they should start by regularly assessing their security risk: what technology is being used, what data is being collected by that technology, and how and where the data is being stored and protected. Once the risks are identified, they should determine risk mitigation strategies, part of which may bring in new tools and processes, such as automated breach detection software. Such software looks for activity patterns that might signal significant threats, e.g., abnormal off-hour activity and abnormal access to data, and has been identified by the IBM study to dramatically decrease the total cost of a data breach: organizations with fully-deployed security automation experienced breach costs that were about half as much of those that didn’t in 2019.
Other known best practices include performing penetration testing to identify and reinforce vulnerable spots and simulating phishing attacks –which the added advantage that it strengthens your staff training. Last but not least, the ability of an organization to respond effectively after a data breach is reinforced by the existence of a breach response team that has crafted a breach response plan and conducted extensive plan testing.

Challenges and recommendations specific to data privacy
In today’s age of data-driven decisions, businesses need to exploit the utility of their consumer data for some business purpose. This is generally done through analytics: training a machine learning model to predict consumer behaviour and better personalize interactions with them, or querying a consumer transaction warehouse to build a report. But they need now to (i) respect data privacy principles present in today’s privacy regulations, (ii) allow for consumers to exercise their privacy rights, and (iii) prevent linkage attacks, that is, reidentification of people, when exploiting the data utility through analytics. All of this builds on top of data security: there’s no data privacy without security!
We briefly mentioned data encryption before; the data security toolkit also includes access control, redaction, masking, and so on. But if businesses apply these techniques too heavily, they might get little or no utility out of their data –at least with today’s available technology. To get the utility companies need, they have to adopt a risk-tolerance mindset, weighing privacy imperatives against using personal data to drive business value. We believe privacy should be treated as a parameter in every initiative that uses personal data. But initiatives such as selecting an audience for a marketing campaign, or detecting suspicious anti-money laundering in a bank’s AML compliance office, have very different privacy parameters and require the understanding of privacy risks specific to each case.
Which brings us to one of the main challenges of data privacy in businesses: obtaining an aligned view of the privacy risks and the tolerance for them. Space needs to be provided where these risks can be discussed. A data governance framework is an example of such a space. Education is key to get a common understanding because privacy risks are not intuitive.

A second challenge I want to mention here (there are others) is that data provisioning processes for initiatives such as the above are frequently manual, custom processes, so they are slow. They are also brittle, hard to audit and prone to linkage attacks. The recommendation is to automate your risk tolerance design into data pipeline processes similar to those you have for data integration and data quality, and delivering secured datasets into your existing data environments, where existing access control techniques can be applied, and where they can be audited internally to control your privacy posture, and by compliance authorities.

Fernando Velez
Fernando Velez is chief data technologist at Persistent Systems. The views in this article are his own.