
AI can come to the rescue of firefighters, say researchers
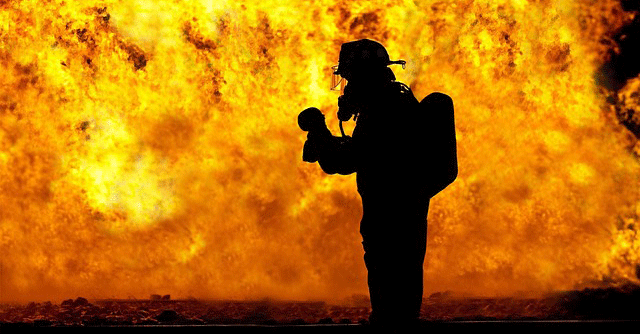

When a building is on fire, it is difficult to notice the signs of impending flashover – a deadly fire phenomenon that can suddenly ignite and burn everything in a room and is a leading cause of firefighter deaths. A new research suggests that artificial intelligence (AI) could forewarn and come to the rescue of firefighters.
The researchers at the National Institute of Standards and Technology (NIST), the Hong Kong Polytechnic University have developed a model, dubbed as Flashover Prediction Neural Network (FlashNet), to forecast the lethal events precious seconds before a real fire erupts and cause the deadly damage.
The new study published in ‘Engineering Applications of Artificial Intelligence’, said that FlashNet has an accuracy of up to 92% across more than a dozen common residential floorplans in the US and overtook other AI-based flashover predicting programmes.

“Until now, most machine learning (ML)-based prediction tools have been trained to operate in a single, familiar environment. In reality, firefighters are not afforded such luxury. As they charge into hostile territory, they may know little to nothing about the floorplan, the location of fire or whether doors are open or closed,” the research paper said.
“Our previous model only had to consider four or five rooms in one layout, but when the layout switches and you have 13 or 14 rooms, it can be a nightmare for the model. For real-world application, we believe the key is to move to a generalised model that works for many different buildings,” Wai Cheong Tam, NIST mechanical engineer, co-first author of the study said.
To cope with the variability of real fires, the researchers improved their approach with graph neural networks (GNN), a kind of ML algorithm good at making judgments based on graphs of nodes and lines, representing different data points and their relationships with one another. GNNs are frequently used for estimated time of arrival (ETA), in traffic that can be used to analyse 10 to 50 different roads.

The researchers digitally simulated more than 41,000 fires in 17 kinds of buildings, representing a majority of the US residential building stock. In addition to layout, factors such as the origin of the fire, types of furniture and whether doors and windows were open or closed varied throughout.
While the tool's performance was quite promising, Tam said, researchers “still have a way to go before they can take FlashNet across the finish line”.
As a next step, the researchers plan to battle-test the model with real-world, rather than simulated, data. "In order to fully test our model's performance, we actually need to build and burn our own structures and include some real sensors in them," Tam said, adding that “at the end of the day, that's a must if we want to deploy this model in real fire scenarios”.
