
Meta’s new AI model can cut out objects in images
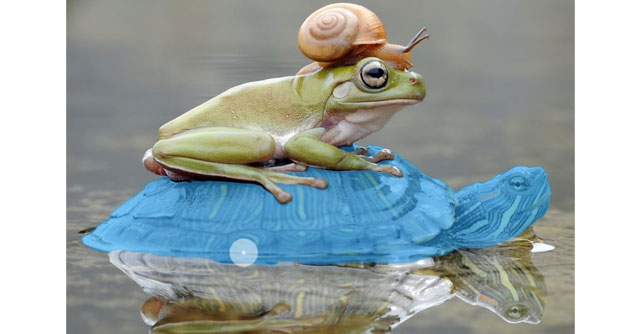

Researchers at Meta Platforms have built a new artificial intelligence (AI) model, called the Segment Anything Model (SAM), which can identify and remove objects from any image using a few mouse clicks. Text prompts have been tested for the model but it has not been released right now, the company added.
According to Meta, SAM has been trained on a dataset, called SA-1B V1.0, which consists of 11 million high-resolution and privacy-protected images, which were licensed from a large photo company. Meta claims that it is the largest segmentation dataset till date.
Meta said it has made this dataset public so it can be used by others for computer vision research and training general-purpose object segmentation models.
Meanwhile, the AI model is available under a permissive open license and can be accessed through a web browser.

In an official blog post published Wednesday, the Segment Anything research team explains that the data required to train a segmentation model is “not readily available” online unlike images, videos, and text, which is easily available.
Based on a transformer vision model, SAM uses an image encoder to map the image features and then uses a set of prompt embeddings to produce a segmentation mask. The mask segments the object that has to be deleted from the image. The team claims SAM can segment an object in just 50 milliseconds after receiving a prompt.
Transformers are neural networks that can understand the link between two sequential data such as words in a sentence or objects in an image. OpenAI’s text-to-image model DALL-E or Stability AI’s Stable Diffusion are some of the AI models that are based on transformers.

Further, researchers at Meta said that SAM was used to annotate images, and then the annotated data was used to update it. “We repeated this cycle many times to iteratively improve both the model and dataset,” the research team added.
However, the team realized that image annotation wasn't sufficient to train a large dataset. So they built a data engine with three processes. In the first process, the model assists annotators, while in the second a combination of automatic and assisted annotation was used. In the third process, the data engine completely automated the mask creation, allowing it to scale to include more than 1.1 billion segmentation masks.
Though the images used for the dataset were geographically diverse and from multiple countries, the researchers acknowledge that certain geographic regions are still underrepresented. Also, to ensure it doesn’t discriminate against any groups, researchers analyzed it for potential biases with respect to gender, skin tone, and age.
