
Cybersecurity is a data problem, security leaders must leverage emerging capabilities: Omer Singer of Snowflake
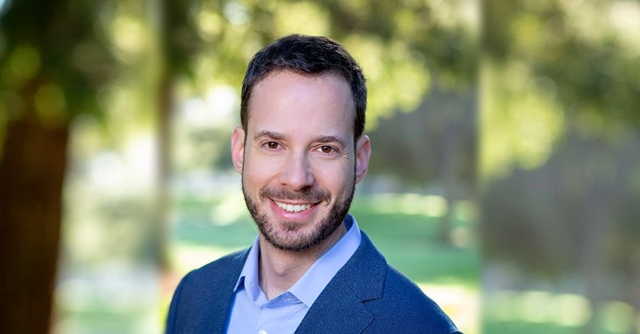

Cybersecurity is at the heart of data cloud company SnowFlake’s core offering. The barely decade-old company commanding $55 billion market cap, Snowflake enables organisations to mobilise their data to unite siloed data, discover and securely share data, power data applications, and execute diverse Artificial Intelligence/Machine Learning (AI/ML) and analytic workloads. In an exclusive interview with TechCircle, Omer Singer, Head of Cybersecurity Strategy at Snowflake, talked about cybersecurity trends, highlighted the importance of security data lakes, and shared insights on the transformative impact of generative AI and large language models (LLMs). Edited excerpts:
What changes have you noticed in the cybersecurity landscape in recent years, particularly after the pandemic? Are there specific trends fuelling these shifts?
A notable trend I find fascinating is the growing recognition that cybersecurity is fundamentally a data challenge. Security teams are now approaching cybersecurity as a data issue, drawing insights from successful practices in other sectors and organisations. They are leveraging innovations from the realm of data, such as the modern data stack, to enhance their security efforts within the organisation. This paradigm shift necessitates security teams to strategically select tools for specific use cases. The key shift lies in integrating diverse data sets, leading to a scenario where cybersecurity solution providers can align their offerings with the unified data source, known as a security data lake. Security data lakes have emerged as an architectural solution that enables security leaders to consolidate security data, regardless of its quantity and diversity. This consolidation facilitates the implementation of genuine accountability measures throughout the organisation. This concept has become a pivotal development in cybersecurity, holding promising prospects for safeguarding enterprises.
Are there any upcoming technologies or practices that will significantly influence the cybersecurity landscape in the future? How is Snowflake positioning itself to tackle these emerging trends?
The topic that's currently dominating discussions is the emergence of generative AI and large language models (LLMs). We are standing at the brink of a paradigm shift; this mirrors the magnitude of the shift to cloud computing. This shift toward generative AI in cybersecurity echoes the lessons learned during the cloud adoption phase. It became evident that traditional methods and risk assessments, designed for on-premise environments, didn't seamlessly translate into cloud-centric infrastructures. Similarly, with the advent of generative AI, it's crucial to evaluate the security implications. We need to consider how these intelligent algorithms are trained, establish trust in their responses, and ensure they haven't been manipulated by threat actors.

Snowflake is committed to helping organisations adopt generative AI technology in a secure and governed manner. We firmly believe that data should remain within the organisation's governance perimeter, eliminating the need for it to be sent to third parties for training or inference. Our approach empowers customers to run their chosen models within the data platform, significantly reducing or eliminating risks. Although challenging, we are heavily investing in this concept, with exciting developments on the horizon regarding running large language models within the Snowflake Data Cloud. This initiative not only focuses on mitigating risks but also presents a unique opportunity.
With cybersecurity increasingly viewed as a data challenge, security leaders are eager to leverage these emerging capabilities to tackle security issues. Already, the power of generative AI is proving invaluable in the realm of security operations. It aids Security Operations Centers (SOCs) in crafting more effective threat detection rules and expedites responses to security incidents by simplifying complex alerts for analysts of varying expertise levels.
How do you perceive the impact of technologies such as AI and ML on cybersecurity? And how does Snowflake utilise these technologies to enhance security measures?
Threat actors are continuously seeking new methods to breach their targets, while defenders are constantly devising strategies to prevent these breaches. The introduction of powerful technologies like generative AI and its easy accessibility to everyone has created a scenario where both sides can utilise these advancements. In the near future, we anticipate threat actors employing generative AI and LLMs to swiftly progress from initial access to complete compromise and achievement of their objectives. Preventing an initial breach is challenging in any case, and now with advanced technology, threat actors can swiftly interpret the initial access points and formulate attack paths, expediting compromise. This rapid transformation poses a significant challenge, as it reduces the time defenders have to prevent a breach.

While hackers are leveraging these technologies, there are also opportunities for SOCs and security teams to explore the application of LLMs to enhance threat detection and respond more efficiently to attacks they identify. Moreover, this advancement presents an exciting prospect for stakeholders within the organisation.
Today, every leader, from the CEO to the CFO and the CRO, is deeply concerned about the organisation's security posture. It's not limited to just the CIO or CISO. A breach impacts the company's valuation and its ability to engage with customers. In the past, discussing the organisation's security status required a certain level of expertise. However, now, individuals, regardless of their skill level, can ask fundamental questions about the enterprise's security. This accessibility enables informed conversations with the security organisation, allowing stakeholders to hold them accountable for the security posture everyone relies on.
Data scarcity in India has been a longstanding concern. What is your opinion on this matter?
When considering the factors contributing to data scarcity, a combination of issues comes to light. One factor could be the substantial investment traditionally required to establish significant big data capabilities; a feat achieved by large multinational companies in the United States. Early pioneers like Yahoo invested billions of dollars to create cutting-edge big data technology at a petabyte scale. However, technologies like Snowflake are now democratising the big data platform. Solutions such as Snowflake make it possible for anyone, without any upfront investment, to access a powerful, resilient, and petabyte-scale data platform, enabling immediate engagement. This accessibility addresses challenges faced by companies and organisations in India.

Moreover, with the integration of LLMs deeply into the data cloud, more diverse groups can participate meaningfully. You no longer need to be a Structured Query Language (SQL) expert to interact with data; simply describing your queries in natural language is sufficient. Questions like sales figures in specific regions or forecasts for the next quarter can now be articulated without intricate technical expertise. Furthermore, fundamental tools to extract insightful information are now delivered as services, democratising access to advanced functions such as anomaly detection. This approach, aimed at democratising the components of the modern data stack, helps overcome the scarcity of data issue.
Lastly, the matter of access to high-quality, up-to-date datasets is crucial. Previously, establishing personal relationships with substantial financial commitments was often the norm to gain access to such data. However, platforms like Snowflake have introduced marketplaces, like the Snowflake Marketplace, where users can readily find the data they need and commence working with it immediately. This approach significantly improves the landscape of data scarcity challenges in India.
Could you provide some information about Snowflake's present and future technological investments, particularly in the field of cybersecurity? Are there any specific areas you are focusing on?
In 2022, Snowflake introduced its cybersecurity workload, marking a significant investment in being committed to making Snowflake the premier data platform for security applications. Recognising security as a data challenge, we understood the need for substantial investments to handle the variety and speed at which security data is generated today. Consequently, we've rolled out numerous innovations and features tailored to support security use cases directly. This effort has cultivated a robust ecosystem where solutions are developed around Snowflake to address complex issues in threat detection, response, compliance automation, and vulnerability management.
Within the cybersecurity industry, these security challenges are being tackled through a network of applications that utilise Snowflake as the backend. Looking ahead, our focus is on how these solutions are deployed.

We anticipate that with our ongoing investments in Snowflake as an application platform, security solutions will increasingly be deployed atop the customer’s Snowflake environment. We believe this approach will unlock additional value, offering enhanced visibility and automation for security teams utilising these advanced cybersecurity solutions within their Snowflake security data lake.
Could you provide details about Snowflake's upcoming offerings related to generative AI?
I am excited about Snowpark and ML; we view them as facilitating open-source LLM deployment in Snowflake. The concept involves taking powerful open-source models like Llama 2 from Meta and swiftly deploying them into a model registry within Snowflake. This deployment utilises a compute pool equipped with Graphics Processing Units (GPUs). One of the significant challenges lies in accessing GPUs, which are typically in limited supply. However, Snowflake is addressing this by providing our customers with easy access to GPUs within the platform. This enables them to train models like Llama 2 efficiently and deploy them for production, running against their real-time data. Our data is continually refreshed with new streaming capabilities such as Snowpipe Streaming, ensuring the data used for these models is always current.
This comprehensive support for the end-to-end machine learning lifecycle within Snowflake, particularly with these exciting capabilities, is noteworthy. We anticipate that these features will be embraced by security operations centers worldwide, enhancing the effectiveness and cost-efficiency of security programs.
