
From words to feelings: How modern LLMs are redefining sentiment analysis
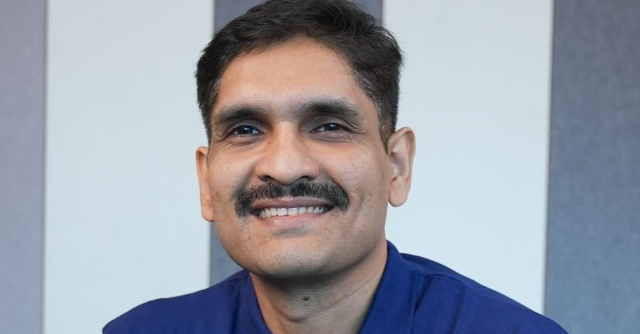

Sentiment analysis once seemed revolutionary — categorize user feedback as positive, negative, or neutral, and you had a clear snapshot of public opinion (sort of). Yet, in an era of hyper-connected social media and evolving human expression, that snapshot now feels too narrow. Subtle cues – such as irony, context-specific slang, or cultural nuances – can flip a seemingly positive statement into a complaint – or vice versa.
Simply put, sentiment analysis is transitioning beyond color-coded bar charts towards a richer understanding of human emotions behind every word. This demands a rethinking of the way we gauge sentiments.
Why do we even need a rethink?
Because the old ways simply don’t cut it anymore. Traditional sentiment analysis worked on a more mechanical level, so to speak, often matching words to a sentiment dictionary to gauge positivity or negativity. While this offered a high-level indicator, it rarely captured the reasons behind those sentiments, missed mixed or nuanced emotions, and struggled with rapidly changing language.

In a MarTech landscape where a single influencer post or viral tweet can reshape brand perception overnight, guessing or oversimplifying sentiment is no longer viable. Deeper, context-driven insight is the new imperative.
So what really changed?
The short answer: modern LLMs. These AI models are trained on massive amounts of text, picking up subtle cues (thanks to their pattern-recognition prowess) that older models might overlook. Based on adequate training data and guidelines, rather than seeing words in isolation, they analyze them within the broader context to figure out the deeper emotional picture.
This shift explains why LLMs interpret complex, multi-layered emotions and detect when praise transitions into dissatisfaction—like reading between the lines of a heartfelt review or realizing that a “sick beat” might actually mean something positive.
Why are LLMs winning hearts (and minds)?

Context, context, context. They don’t just parse words; they sense relationships, intentions, and surrounding ideas that shape meaning.And when new slang trends on social media, they adapt in real time—staying current with ever-evolving language.
What gives LLM-powered sentiment analysis an edge above all is that it offers answers at a granular level, flagging subtle shifts and trending frustrations before they escalate into PR nightmares. For instance, a surge in reviews saying ‘The price is high but so is durability’ could reflect mixed sentiment—negative for price, positive for product quality. Instead of simply categorizing the comment as negative, brands can pinpoint root causes and help marketers optimize messaging accordingly. With precise insights, responses become personal, empathetic, and ultimately more effective in preserving brand loyalty.
The struggles behind the scenes
Rewriting your sentiment analysis from scratch or integrating an LLM is not as simple as flipping a switch. Here are some challenges along the way:

Bias in AI Models: LLMs learn from massive datasets—some of which might be skewed, underrepresent certain groups, or include hidden biases. Without careful monitoring, these biases seep into sentiment analysis and misinterpret real-world conversations. Regular audits and diverse training data become non-negotiable to keep your AI fair and balanced.
Computational and Cost Constraints: Running a giant LLM is no casual affair. You’re dealing with heavy compute loads, specialized hardware, or cloud servers that can rack up expenses. Smaller companies might need to find creative, scaled-down implementations or specialized models to avoid burning a hole in the budget.
Ethical AI and Privacy Concerns: Sentiment analysis dives into people’s emotions—and that’s delicate ground. Collecting and interpreting personal data requires strict compliance with privacy laws (like DPDP, CPA, GDPR, etc.) and a strong ethical backbone. Overstep, and you risk losing trust faster than you can say “data breach.”
The Future of Sentiment Analysis with AI

We’re moving beyond just text. Tomorrow’s sentiment analysis could integrate voice tonality or even facial expressions (where permissible), offering marketers a 360-degree view of how audiences truly feel. Transcending to modern LLM-based sentiment analysis, combined with other AI modalities, will elevate the experience of sentiment analysis for marketers and help them with deeper and actionable insights to connect with their audience. Imagine a chatbot that senses your impatience and responds with a quick resolution and a dash of humor rather than a generic “Our team is looking into it.” AI-powered empathy can deepen customer relationships, showing people they’re not just talking to a bot but a system that “gets” them at a human level.
Eventually, LLMs won’t just gauge current sentiment;they’ll map emotions to specific product or service aspects and anticipate how those sentiments might evolve. Anticipating a future dip in satisfaction could let you remedy the issues in advance, turning potential crises into opportunities for delight. It’s like having a crystal ball for customer perception—minus the mystic vibes and guesswork.
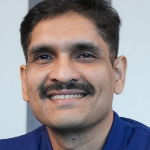
Jacob Joseph
Jacob Joseph is VP - Data Science at CleverTap.