
Microsoft says its Chinese-to-English translator approaches human efficacy
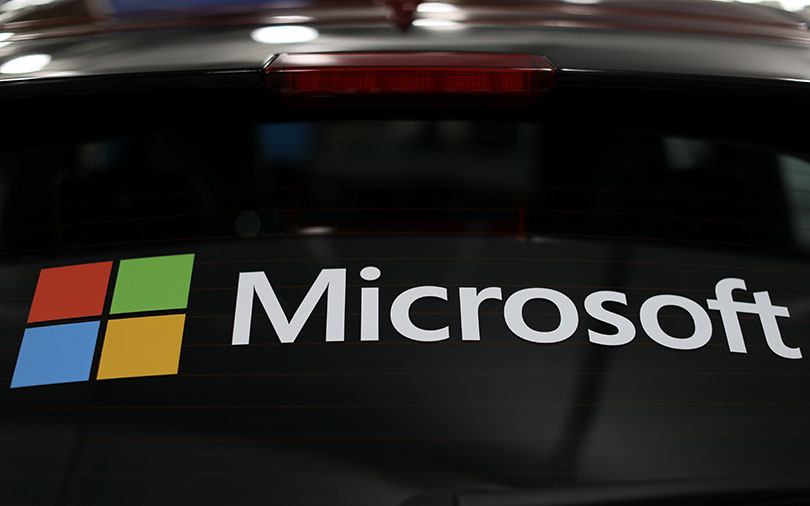

Tech giant Microsoft Corp. says it has built a system that can translate news articles from Chinese to English and vice versa, just as a human would, in what it calls a breakthrough.
The translator mimics how people improve their work, by going over it again and again until they get it right.
One human method used to build the system is called dual learning. Every time Microsoft researchers sent a sentence through the system to be translated from Chinese to English, they also translated it back from English to Chinese. That’s similar to what people might do to make sure their automated translations were accurate. This method allowed the system to learn from its own mistakes.

Another method, called deliberation networks, is similar to how people edit and revise their own writing by going through it again and again. Under this method, researchers taught the system to keep translating the same sentence until better output is achieved.
Under another technique called joint training, the English-to-Chinese system translates new English sentences into Chinese to obtain new sentence pairs. Those are then added to the training data set that is going in the opposite direction, that is, from Chinese to English. The same procedure is then carried out in the other direction, and this ping pong continues, improving both systems.
Agreement regularisation is yet another technique. With this, the translation can be generated by having the system read left to right or right to left. If both ways generate the same translation, the result is considered trustworthy.

Rival Google has also been working on breakthroughs in translation systems. It has Google Translate products for the web, smartphone app and the camera, which can translate the writing it sees.
Only last week, a top executive said Google is working on a new translation and machine learning model for languages, with limited or no data sets, to train the neural engines that handle artificial intelligence tasks.
"This is particularly exciting for Indian languages, for which we face a severe shortage of data," said Barak Turovsky, head of product and design at Google Translate and Machine Learning. "As a result, we have achieved a pretty amazing improvement for Indian and other languages, and are working on expanding this approach to more languages and use cases."

Typically, languages for which a translator has a lot of data can be easily worked upon but the problem arises in case of limited data. The data sets the translator works on comprise of translations done by people.