
India needs to prioritise on setting up a sovereign GPU cloud
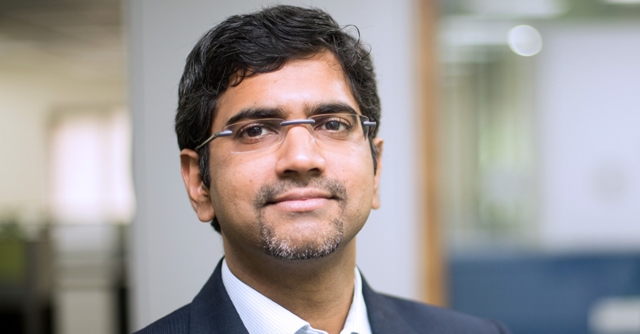

The majority of current large language models (LLMs) originate from the US, lacking representation of diverse languages and cultures. With only 125 million English speakers in India, accounting for less than 10% of the total population, an indigenous LLM for local languages, becomes imperative. In an exclusive interview with TechCircle, S Anjani Kumar, Partner, Consulting, Deloitte India, explains how local language LLMs not only improves digital literacy and foster greater inclusivity, but also ensures better control over data and security. Additionally, Kumar discusses the challenges India is facing in terms of scaling the AI and GPU compute infrastructure. He also sheds light on how countries across the globe are developing culturally relevant AI models that are trained in different local languages to cater to non-English speakers. Edited excerpts:
What challenges does India face in terms of scaling the AI and GPU compute infrastructure compared to global leaders, and what steps are needed to bridge this gap?
India is facing an uphill battle to catch up with global leaders like the United States when it comes to GPU compute infrastructure. The success of Gen AI depends on three pillars — chip manufacturers who provide the required computing power, GPU cloud service providers, and foundational models or large language models. Currently, India lacks in all these aspects. To make an impact and bridge the gap, they should align on the need for a sovereign GPU compute infrastructure to enable the growth of Generative AI locally. They should review engagement models for developing computing infrastructure, for example, hybrid or PPP models, and select the most viable option. There is also a need to build the right partnerships with leading solution providers to get access to the latest GPU computing hardware and software. At the same time, initiating small-scale pilot projects showcasing the potential of Generative AI, working with developers, and developing use cases of indigenous foundation models across the spectrum, from government ministries to public and private industries to the social sector, are also necessary steps.

As LLMs are becoming more popular, what kind of concerns arise pertaining to data privacy, and how can companies ensure training of new models?
With the rapid rise and spread of publicly available LLMs, there have been rising concerns regarding the data privacy and security of user data. Any queries asked, information shared, or data generated can potentially be used by the model to train, re-train, and update itself. Some companies have opted to prohibit their employees from using generative AI chatbots due to apprehensions surrounding the potential exposure of confidential information and have raised concerns about the risk of confidential information inadvertently finding its way onto external servers, prompting them to prioritise safeguarding their proprietary and confidential data.
Organisations with sophisticated technology teams could proceed with Private LLMs and put appropriate guardrails in place, such as training within secure in-house data centres or single tenant private cloud servers, training LLMs using internal workforce, establishing privacy and security controls, thorough model testing to mitigate potential bias, and launching governance programs to prevent model drift, establish trust, and promote safe and responsible use of the output generated through the model. Once the guardrails are in place, wherein models are trained with your data, by your people, in your environment, the risks are substantially mitigated, and the benefits are exponentially manifested.

How according to you can organisations ensure the responsible use of AI?
Since LLMs are not engineered to give a specific result, traditional governance and assurance practices cannot be applied in the same way. Given this, organisations must approach their usage with caution and responsibility, and this is where Trustworthy AI comes into the picture. Trustworthy AI is all about concentrating on the accountable, fair, transparent, and explainable use of AI to achieve desired results. The seven dimensions of Trustworthy AI are transparent and explainable, fair and impartial, robust and reliable, respectful of privacy, safe and secure, and responsible and accountable. Establishing guidelines and regulations for the ethical development and deployment of LLMs is necessary to address issues related to data privacy, security, and potential biases in the output. The Trustworthy AI framework also aims to help businesses increase brand equity and trust, which can lead to new customers, employee retention, and more customers opting to share data. Other potential benefits include increased revenue and reduced costs through more accurate decision-making due to better data sources and reduced legal and remediation costs.
How are collaborations with AI research institutions and domain specialists contributing to the development of specialised LLMs in various sectors as part of India's AI ambitions?

Collaboration with AI research institutions, academia, and domain experts is helping contribute to designing language-specific architecture tailored to local language intricacies and catering to specific industries such as healthcare, education, finance, legal systems, etc. with their own terminologies and requirements. For example, some of the top engineering colleges in India are involved in projects to understand how technology can help create tools similar to OpenAI’s ChatGPT, but in Indian languages or for specific purposes. The Indian Institute of Technology (IIT) Guwahati is working on creating “affordable visual animation models that study eyes and facial movements from open-source visual databases”. IIT Delhi has created a language model called ‘MatSciBert’ specifically for the field of material science research. AI4Bharat, a research lab at IIT Madras, has been at the forefront of building Indic large language models. Furthermore, domain experts in Google AI Research Lab in Bengaluru are developing a multi-lingual AI model to support 100+ Indian languages, which will be integrated into Google products including Bard. Wipro is also engaged with the AI Institute at the University of South Carolina and IIT Patna to build domain-specific language models. These initiatives are aimed at accelerating medical research and providing better medical care.
What engagement model could India adopt to meet its AI ambitions?
India needs to prioritise the establishment of a Sovereign GPU Cloud by acquiring and maintaining the required GPU infrastructure in collaboration with the industry. The optimal mode of engagement should be adapted and contextualised to the total cost of ownership, data access, and data security needs of Gen AI in the Indian context.

Countries worldwide are deploying different engagement models for scaling their sovereign GPU infrastructure. For instance, US’ investment in GPU compute infrastructure is primarily driven by private companies. The Government of Japan, on the other hand, is developing sovereign GPU clouds in collaboration with the industry. Furthermore, the UAE, Saudi Arabia, and the United Kingdom are scaling up through state-owned entities in collaboration with GPU OEMs. These can be private-led, hybrid or PPP models, wherein partial to full initial investment is made by the government while operations, maintenance, and hosting are done by private cloud service providers. They can also be government-led or in-house, where the infrastructure is set up, operated, maintained, and hosted by the government. With the various available options, there is no single "best" answer for India. While the initial investments in GPU infrastructure can be led by the government, in the long term, India can explore private sector participation to scale up the GPU infrastructure. Given the large scale of CAPEX and OPEX investments involved, these projects need to be supported with strategically planned long-term incentive programs to accelerate industry investment in the core building blocks of the AI stack: data, computing, models, applications, and services, and talent – to spur growth across the broader AI ecosystem, including start-ups and academia.